Open Access
ARTICLE
A Shared Natural Neighbors Based-Hierarchical Clustering Algorithm for Discovering Arbitrary-Shaped Clusters
College of Computer and Information Science, Chongqing Normal University, Chongqing, 400030, China
* Corresponding Author: Ji Feng. Email:
(This article belongs to the Special Issue: Advanced Data Mining Techniques: Security, Intelligent Systems and Applications)
Computers, Materials & Continua 2024, 80(2), 2031-2048. https://doi.org/10.32604/cmc.2024.052114
Received 23 March 2024; Accepted 14 June 2024; Issue published 15 August 2024
Abstract
In clustering algorithms, the selection of neighbors significantly affects the quality of the final clustering results. While various neighbor relationships exist, such as K-nearest neighbors, natural neighbors, and shared neighbors, most neighbor relationships can only handle single structural relationships, and the identification accuracy is low for datasets with multiple structures. In life, people’s first instinct for complex things is to divide them into multiple parts to complete. Partitioning the dataset into more sub-graphs is a good idea approach to identifying complex structures. Taking inspiration from this, we propose a novel neighbor method: Shared Natural Neighbors (SNaN). To demonstrate the superiority of this neighbor method, we propose a shared natural neighbors-based hierarchical clustering algorithm for discovering arbitrary-shaped clusters (HC-SNaN). Our algorithm excels in identifying both spherical clusters and manifold clusters. Tested on synthetic datasets and real-world datasets, HC-SNaN demonstrates significant advantages over existing clustering algorithms, particularly when dealing with datasets containing arbitrary shapes.Keywords
Cite This Article
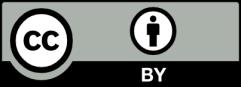
This work is licensed under a Creative Commons Attribution 4.0 International License , which permits unrestricted use, distribution, and reproduction in any medium, provided the original work is properly cited.