Open Access
ARTICLE
Adaptive Graph Convolutional Adjacency Matrix Network for Video Summarization
School of Cyberspace Security, Gansu University of Political Science and Law, Lanzhou, 730000, China
* Corresponding Author: Jing Zhang. Email:
Computers, Materials & Continua 2024, 80(2), 1947-1965. https://doi.org/10.32604/cmc.2024.051781
Received 15 March 2024; Accepted 11 June 2024; Issue published 15 August 2024
Abstract
Video summarization aims to select key frames or key shots to create summaries for fast retrieval, compression, and efficient browsing of videos. Graph neural networks efficiently capture information about graph nodes and their neighbors, but ignore the dynamic dependencies between nodes. To address this challenge, we propose an innovative Adaptive Graph Convolutional Adjacency Matrix Network (TAMGCN), leveraging the attention mechanism to dynamically adjust dependencies between graph nodes. Specifically, we first segment shots and extract features of each frame, then compute the representative features of each shot. Subsequently, we utilize the attention mechanism to dynamically adjust the adjacency matrix of the graph convolutional network to better capture the dynamic dependencies between graph nodes. Finally, we fuse temporal features extracted by Bi-directional Long Short-Term Memory network with structural features extracted by the graph convolutional network to generate high-quality summaries. Extensive experiments are conducted on two benchmark datasets, TVSum and SumMe, yielding F1-scores of 60.8% and 53.2%, respectively. Experimental results demonstrate that our method outperforms most state-of-the-art video summarization techniques.Keywords
Cite This Article
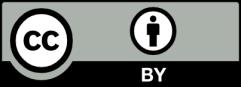
This work is licensed under a Creative Commons Attribution 4.0 International License , which permits unrestricted use, distribution, and reproduction in any medium, provided the original work is properly cited.