Open Access
ARTICLE
Research on Improved MobileViT Image Tamper Localization Model
1 School of Computer Science and Technology, Xi’an University of Posts and Telecommunications, Xi’an, 710121, China
2 Shaanxi Key Laboratory of Network Data Analysis and Intelligent Processing, Xi’an University of Posts and Telecommunications, Xi’an, 710121, China
* Corresponding Author: Fengling Zhang. Email:
Computers, Materials & Continua 2024, 80(2), 3173-3192. https://doi.org/10.32604/cmc.2024.051705
Received 12 March 2024; Accepted 18 July 2024; Issue published 15 August 2024
Abstract
As image manipulation technology advances rapidly, the malicious use of image tampering has alarmingly escalated, posing a significant threat to social stability. In the realm of image tampering localization, accurately localizing limited samples, multiple types, and various sizes of regions remains a multitude of challenges. These issues impede the model’s universality and generalization capability and detrimentally affect its performance. To tackle these issues, we propose FL-MobileViT-an improved MobileViT model devised for image tampering localization. Our proposed model utilizes a dual-stream architecture that independently processes the RGB and noise domain, and captures richer traces of tampering through dual-stream integration. Meanwhile, the model incorporating the Focused Linear Attention mechanism within the lightweight network (MobileViT). This substitution significantly diminishes computational complexity and resolves homogeneity problems associated with traditional Transformer attention mechanisms, enhancing feature extraction diversity and improving the model’s localization performance. To comprehensively fuse the generated results from both feature extractors, we introduce the ASPP architecture for multi-scale feature fusion. This facilitates a more precise localization of tampered regions of various sizes. Furthermore, to bolster the model’s generalization ability, we adopt a contrastive learning method and devise a joint optimization training strategy that leverages fused features and captures the disparities in feature distribution in tampered images. This strategy enables the learning of contrastive loss at various stages of the feature extractor and employs it as an additional constraint condition in conjunction with cross-entropy loss. As a result, overfitting issues are effectively alleviated, and the differentiation between tampered and untampered regions is enhanced. Experimental evaluations on five benchmark datasets (IMD-20, CASIA, NIST-16, Columbia and Coverage) validate the effectiveness of our proposed model. The meticulously calibrated FL-MobileViT model consistently outperforms numerous existing general models regarding localization accuracy across diverse datasets, demonstrating superior adaptability.Keywords
Cite This Article
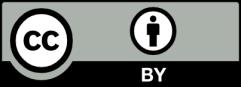
This work is licensed under a Creative Commons Attribution 4.0 International License , which permits unrestricted use, distribution, and reproduction in any medium, provided the original work is properly cited.