Open Access
ARTICLE
HybridGAD: Identification of AI-Generated Radiology Abstracts Based on a Novel Hybrid Model with Attention Mechanism
1 Department of Software Engineering, Faculty of Technology, Manisa Celal Bayar University, Manisa, 45140, Turkey
2 Department of Computer Engineering, Faculty of Engineering and Architecture, İzmir Katip Çelebi University, İzmir, 35620, Turkey
* Corresponding Author: Aytuğ Onan. Email:
Computers, Materials & Continua 2024, 80(2), 3351-3377. https://doi.org/10.32604/cmc.2024.051574
Received 09 March 2024; Accepted 24 June 2024; Issue published 15 August 2024
Abstract
The purpose of this study is to develop a reliable method for distinguishing between AI-generated, paraphrased, and human-written texts, which is crucial for maintaining the integrity of research and ensuring accurate information flow in critical fields such as healthcare. To achieve this, we propose HybridGAD, a novel hybrid model that combines Long Short-Term Memory (LSTM), Bidirectional LSTM (Bi-LSTM), and Bidirectional Gated Recurrent Unit (Bi-GRU) architectures with an attention mechanism. Our methodology involves training this hybrid model on a dataset of radiology abstracts, encompassing texts generated by AI, paraphrased by AI, and written by humans. The major findings of our analysis indicate that HybridGAD achieves a high accuracy of 98%, significantly outperforming existing state-of-the-art models. This high performance is attributed to the model’s ability to effectively capture the contextual nuances and structural differences between AI-generated and human-written texts. In conclusion, HybridGAD not only enhances the accuracy of text classification in the field of radiology but also paves the way for more advanced medical diagnostic processes by ensuring the authenticity of textual information. Future research will focus on integrating textual and visual data for comprehensive radiology assessments and improving model generalization with partially labeled data. This study underscores the potential of HybridGAD in transforming medical text classification and highlights its applicability in ensuring the integrity and reliability of research in healthcare and beyond.Keywords
Cite This Article
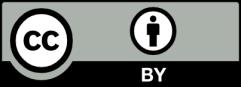
This work is licensed under a Creative Commons Attribution 4.0 International License , which permits unrestricted use, distribution, and reproduction in any medium, provided the original work is properly cited.