Open Access
ARTICLE
Dynamic Offloading and Scheduling Strategy for Telematics Tasks Based on Latency Minimization
1 North China Institute of Computing Technology Beijing, Beijing, 100080, China
2 School of Computer and Communication Engineering, University of Science and Technology Beijing, Beijing, 100083, China
* Corresponding Author: Yueqiang Xu. Email:
(This article belongs to the Special Issue: Advanced Communication and Networking Technologies for Internet of Things and Internet of Vehicles)
Computers, Materials & Continua 2024, 80(2), 1809-1829. https://doi.org/10.32604/cmc.2024.050975
Received 24 February 2024; Accepted 28 April 2024; Issue published 15 August 2024
Abstract
In current research on task offloading and resource scheduling in vehicular networks, vehicles are commonly assumed to maintain constant speed or relatively stationary states, and the impact of speed variations on task offloading is often overlooked. It is frequently assumed that vehicles can be accurately modeled during actual motion processes. However, in vehicular dynamic environments, both the tasks generated by the vehicles and the vehicles’ surroundings are constantly changing, making it difficult to achieve real-time modeling for actual dynamic vehicular network scenarios. Taking into account the actual dynamic vehicular scenarios, this paper considers the real-time non-uniform movement of vehicles and proposes a vehicular task dynamic offloading and scheduling algorithm for single-task multi-vehicle vehicular network scenarios, attempting to solve the dynamic decision-making problem in task offloading process. The optimization objective is to minimize the average task completion time, which is formulated as a multi-constrained non-linear programming problem. Due to the mobility of vehicles, a constraint model is applied in the decision-making process to dynamically determine whether the communication range is sufficient for task offloading and transmission. Finally, the proposed vehicular task dynamic offloading and scheduling algorithm based on muti-agent deep deterministic policy gradient (MADDPG) is applied to solve the optimal solution of the optimization problem. Simulation results show that the algorithm proposed in this paper is able to achieve lower latency task computation offloading. Meanwhile, the average task completion time of the proposed algorithm in this paper can be improved by 7.6% compared to the performance of the MADDPG scheme and 51.1% compared to the performance of deep deterministic policy gradient (DDPG).Keywords
Cite This Article
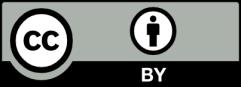
This work is licensed under a Creative Commons Attribution 4.0 International License , which permits unrestricted use, distribution, and reproduction in any medium, provided the original work is properly cited.