Open Access
ARTICLE
A Hybrid Deep Learning and Machine Learning-Based Approach to Classify Defects in Hot Rolled Steel Strips for Smart Manufacturing
Department of Information and Communication Engineering, Changwon National University, Changwon, 51140, Republic of Korea
* Corresponding Authors: Jungpyo Hong. Email: ; Jongwon Seok. Email:
(This article belongs to the Special Issue: Advanced Artificial Intelligence and Machine Learning Frameworks for Signal and Image Processing Applications)
Computers, Materials & Continua 2024, 80(2), 2099-2119. https://doi.org/10.32604/cmc.2024.050884
Received 21 February 2024; Accepted 20 June 2024; Issue published 15 August 2024
Abstract
Smart manufacturing is a process that optimizes factory performance and production quality by utilizing various technologies including the Internet of Things (IoT) and artificial intelligence (AI). Quality control is an important part of today’s smart manufacturing process, effectively reducing costs and enhancing operational efficiency. As technology in the industry becomes more advanced, identifying and classifying defects has become an essential element in ensuring the quality of products during the manufacturing process. In this study, we introduce a CNN model for classifying defects on hot-rolled steel strip surfaces using hybrid deep learning techniques, incorporating a global average pooling (GAP) layer and a machine learning-based SVM classifier, with the aim of enhancing accuracy. Initially, features are extracted by the VGG19 convolutional block. Then, after processing through the GAP layer, the extracted features are fed to the SVM classifier for classification. For this purpose, we collected images from publicly available datasets, including the Xsteel surface defect dataset (XSDD) and the NEU surface defect (NEU-CLS) datasets, and we employed offline data augmentation techniques to balance and increase the size of the datasets. The outcome of experiments shows that the proposed methodology achieves the highest metrics score, with 99.79% accuracy, 99.80% precision, 99.79% recall, and a 99.79% F1-score for the NEU-CLS dataset. Similarly, it achieves 99.64% accuracy, 99.65% precision, 99.63% recall, and a 99.64% F1-score for the XSDD dataset. A comparison of the proposed methodology to the most recent study showed that it achieved superior results as compared to the other studies.Keywords
Cite This Article
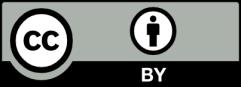
This work is licensed under a Creative Commons Attribution 4.0 International License , which permits unrestricted use, distribution, and reproduction in any medium, provided the original work is properly cited.