Open Access
ARTICLE
Squeeze and Excitation Convolution with Shortcut for Complex Plasma Image Recognition
1 College of Information and Electrical Engineering, China Agricultural University, Beijing, 100083, China
2 College of Science, China Agricultural University, Beijing, 100083, China
* Corresponding Author: Feng Huang. Email:
Computers, Materials & Continua 2024, 80(2), 2221-2236. https://doi.org/10.32604/cmc.2024.049862
Received 20 January 2024; Accepted 11 May 2024; Issue published 15 August 2024
Abstract
Complex plasma widely exists in thin film deposition, material surface modification, and waste gas treatment in industrial plasma processes. During complex plasma discharge, the configuration, distribution, and size of particles, as well as the discharge glow, strongly depend on discharge parameters. However, traditional manual diagnosis methods for recognizing discharge parameters from discharge images are complicated to operate with low accuracy, time-consuming and high requirement of instruments. To solve these problems, by combining the two mechanisms of attention mechanism (strengthening the extraction of the channel feature) and shortcut connection (enabling the input information to be directly transmitted to deep networks and avoiding the disappearance or explosion of gradients), the network of squeeze and excitation convolution with shortcut (SECS) for complex plasma image recognition is proposed to effectively improve the model performance. The results show that the accuracy, precision, recall and F1-Score of our model are superior to other models in complex plasma image recognition, and the recognition accuracy reaches 97.38%. Moreover, the recognition accuracy for the Flowers and Chest X-ray publicly available data sets reaches 97.85% and 98.65%, respectively, and our model has robustness. This study shows that the proposed model provides a new method for the diagnosis of complex plasma images and also provides technical support for the application of plasma in industrial production.Keywords
Cite This Article
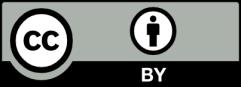
This work is licensed under a Creative Commons Attribution 4.0 International License , which permits unrestricted use, distribution, and reproduction in any medium, provided the original work is properly cited.