Open Access
ARTICLE
Fake News Detection Based on Cross-Modal Message Aggregation and Gated Fusion Network
1 College of Computer, Zhongyuan University of Technology, Zhengzhou, 450007, China
2 Henan Key Laboratory of Cyberspace Situation Awareness, Zhengzhou, 450001, China
* Corresponding Author: Fangfang Shan. Email:
Computers, Materials & Continua 2024, 80(1), 1521-1542. https://doi.org/10.32604/cmc.2024.053937
Received 13 May 2024; Accepted 12 June 2024; Issue published 18 July 2024
Abstract
Social media has become increasingly significant in modern society, but it has also turned into a breeding ground for the propagation of misleading information, potentially causing a detrimental impact on public opinion and daily life. Compared to pure text content, multmodal content significantly increases the visibility and share ability of posts. This has made the search for efficient modality representations and cross-modal information interaction methods a key focus in the field of multimodal fake news detection. To effectively address the critical challenge of accurately detecting fake news on social media, this paper proposes a fake news detection model based on cross-modal message aggregation and a gated fusion network (MAGF). MAGF first uses BERT to extract cumulative textual feature representations and word-level features, applies Faster Region-based Convolutional Neural Network (Faster R-CNN) to obtain image objects, and leverages ResNet-50 and Visual Geometry Group-19 (VGG-19) to obtain image region features and global features. The image region features and word-level text features are then projected into a low-dimensional space to calculate a text-image affinity matrix for cross-modal message aggregation. The gated fusion network combines text and image region features to obtain adaptively aggregated features. The interaction matrix is derived through an attention mechanism and further integrated with global image features using a co-attention mechanism to produce multimodal representations. Finally, these fused features are fed into a classifier for news categorization. Experiments were conducted on two public datasets, Twitter and Weibo. Results show that the proposed model achieves accuracy rates of 91.8% and 88.7% on the two datasets, respectively, significantly outperforming traditional unimodal and existing multimodal models.Keywords
Cite This Article
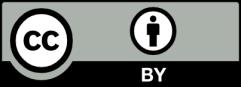
This work is licensed under a Creative Commons Attribution 4.0 International License , which permits unrestricted use, distribution, and reproduction in any medium, provided the original work is properly cited.