Open Access
ARTICLE
A Hybrid Feature Fusion Traffic Sign Detection Algorithm Based on YOLOv7
1 School of Mechanical Engineering, Henan University of Science and Technology, Luoyang, 471000, China
2 School of Information Engineering, Henan University of Science and Technology, Luoyang, 471000, China
3 School of Electronic Information, Zhengzhou Institute of Aeronautical Industry Management, Zhengzhou, 450046, China
4 Henan Province New Energy Vehicle Power Electronics and Power Transmission Engineering Research Center, Luoyang, 471000, China
* Corresponding Author: Juwei Zhang. Email:
(This article belongs to the Special Issue: Metaheuristics, Soft Computing, and Machine Learning in Image Processing and Computer Vision)
Computers, Materials & Continua 2024, 80(1), 1425-1440. https://doi.org/10.32604/cmc.2024.052667
Received 10 April 2024; Accepted 11 June 2024; Issue published 18 July 2024
Abstract
Autonomous driving technology has entered a period of rapid development, and traffic sign detection is one of the important tasks. Existing target detection networks are difficult to adapt to scenarios where target sizes are seriously imbalanced, and traffic sign targets are small and have unclear features, which makes detection more difficult. Therefore, we propose a Hybrid Feature Fusion Traffic Sign detection algorithm based on YOLOv7 (HFFT-YOLO). First, a self-attention mechanism is incorporated at the end of the backbone network to calculate feature interactions within scales; Secondly, the cross-scale fusion part of the neck introduces a bottom-up multi-path fusion method. Design reuse paths at the end of the neck, paying particular attention to cross-scale fusion of high-level features. In addition, we found the appropriate channel width through a lot of experiments and reduced the superfluous parameters. In terms of training, a new regression loss CMPDIoU is proposed, which not only considers the problem of loss degradation when the aspect ratio is the same but the width and height are different, but also enables the penalty term to dynamically change at different scales. Finally, our proposed improved method shows excellent results on the TT100K dataset. Compared with the baseline model, without increasing the number of parameters and computational complexity, and increased by 2.2% and 2.7%, respectively, reaching 92.9% and 58.1%.Keywords
Cite This Article
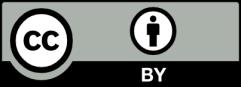
This work is licensed under a Creative Commons Attribution 4.0 International License , which permits unrestricted use, distribution, and reproduction in any medium, provided the original work is properly cited.