Open Access
ARTICLE
EDU-GAN: Edge Enhancement Generative Adversarial Networks with Dual-Domain Discriminators for Inscription Images Denoising
1 School of Mechanical and Precision Instrument Engineering, Xi’an University of Technology, Xi’an, 710048, China
2 Department of Information Science, Xi’an University of Technology, Xi’an, 710054, China
3 School of Faculty of Painting, Packaging Engineering and Digital Media, Xi’an University of Technology, Xi’an, 710048, China
4 School of Computer Science and Engineering, Xi’an University of Technology, Xi’an, 710048, China
* Corresponding Author: Erhu Zhang. Email:
Computers, Materials & Continua 2024, 80(1), 1633-1653. https://doi.org/10.32604/cmc.2024.052611
Received 08 April 2024; Accepted 12 June 2024; Issue published 18 July 2024
Abstract
Recovering high-quality inscription images from unknown and complex inscription noisy images is a challenging research issue. Different from natural images, character images pay more attention to stroke information. However, existing models mainly consider pixel-level information while ignoring structural information of the character, such as its edge and glyph, resulting in reconstructed images with mottled local structure and character damage. To solve these problems, we propose a novel generative adversarial network (GAN) framework based on an edge-guided generator and a discriminator constructed by a dual-domain U-Net framework, i.e., EDU-GAN. Unlike existing frameworks, the generator introduces the edge extraction module, guiding it into the denoising process through the attention mechanism, which maintains the edge detail of the restored inscription image. Moreover, a dual-domain U-Net-based discriminator is proposed to learn the global and local discrepancy between the denoised and the label images in both image and morphological domains, which is helpful to blind denoising tasks. The proposed dual-domain discriminator and generator for adversarial training can reduce local artifacts and keep the denoised character structure intact. Due to the lack of a real-inscription image, we built the real-inscription dataset to provide an effective benchmark for studying inscription image denoising. The experimental results show the superiority of our method both in the synthetic and real-inscription datasets.Keywords
Cite This Article
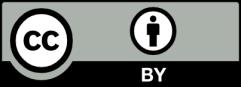