Open Access
ARTICLE
Evaluation of Industrial IoT Service Providers with TOPSIS Based on Circular Intuitionistic Fuzzy Sets
Industrial Engineering Department, Fenerbahçe University, İstanbul, 34758, Turkey
* Corresponding Author: Elif Çaloğlu Büyükselçuk. Email:
Computers, Materials & Continua 2024, 80(1), 715-746. https://doi.org/10.32604/cmc.2024.052509
Received 04 April 2024; Accepted 23 May 2024; Issue published 18 July 2024
Abstract
Industrial Internet of Things (IIoT) service providers have become increasingly important in the manufacturing industry due to their ability to gather and process vast amounts of data from connected devices, enabling manufacturers to improve operational efficiency, reduce costs, and enhance product quality. These platforms provide manufacturers with real-time visibility into their production processes and supply chains, allowing them to optimize operations and make informed decisions. In addition, IIoT service providers can help manufacturers create new revenue streams through the development of innovative products and services and enable them to leverage the benefits of emerging technologies such as Artificial Intelligence (AI) and machine learning. Overall, the implementation of IIoT platforms in the manufacturing industry is crucial for companies seeking to remain competitive and meet the ever-increasing demands of customers in the digital age. In this study, the evaluation criteria to be considered in the selection of IIoT service provider in small and medium-sized (SME) manufacturing enterprises will be determined and IIoT service providers alternatives will be evaluated using the technique for order preference by similarity to an ideal solution (TOPSIS) method based on circular intuitionistic fuzzy sets. Based on the assessments conducted in accordance with the literature review and expert consultations, a set of 8 selection criteria has been established. These criteria encompass industry expertise, customer support, flexibility and scalability, security, cost-effectiveness, reliability, data analytics, as well as compatibility and usability. Upon evaluating these criteria, it was observed that the security criterion holds the highest significance, succeeded by cost-effectiveness, data analytics, flexibility and scalability, reliability, and customer support criteria, in descending order of importance. Following the evaluation of seven distinct alternatives against these criteria, it was deduced that the A6 alternative, a German service provider, emerged as the most favorable option. The identical issue was addressed utilizing sensitivity analysis alongside various multi-criteria decision-making (MCDM) methods, and after comprehensive evaluation, the outcomes were assessed. Spearman’s correlation coefficient was computed to ascertain the association between the rankings derived from solving the problem using diverse MCDM methods.Keywords
The Internet of Things (IoT) emerges as a transformative phenomenon that unites the realms of physical and digital domains through the interconnection of various entities such as devices, sensors, systems, and individuals. This connectivity fosters seamless communication, data sharing, and enhanced operational efficiency. Across diverse sectors including agriculture, healthcare, manufacturing, and transportation, IoT facilitates streamlined business processes and stimulates innovation. Leveraging real-time data, IoT enables the optimization of resource utilization and the automation of tasks, thereby promoting effectiveness and efficiency. Furthermore, the utilization of IoT-generated data empowers businesses to expedite decision-making processes with heightened accuracy. Moreover, IoT exhibits considerable potential in bolstering sustainability initiatives, particularly through the monitoring of energy consumption and waste management, consequently fostering the establishment of environmentally conscious practices within businesses.
The Internet of Things (IoT) is considered one of the foundational technologies driving digital transformation reflecting processes. At its core, IoT is founded on the principle that objects embedded with sensors continually gather information about their surroundings and transmit this data to other devices. This information is evaluated, and subsequent actions are based on this analysis [1–3]. In simple terms, IoT technology can be defined as the connection between people, computers, and objects. This is achieved by using a variety of information sensing equipment, including infrared sensors, Radio Frequency Identification (RFID), laser systems, global positioning systems, and others, to link any item to the Internet protocol (IP) and assign a unique IP address for information exchange and communication. This enables the management, tracking, positioning, and monitoring of items [4].
Over the past decade, the economic potential of the IoT has rapidly become a reality. The global market for IoT services has seen continuous growth and is projected to reach USD 172.6 billion by 2025. The adoption of IoT services has had a significant impact on transforming enterprise business into digital and identifying new business models. Moreover, it has also led to improved productivity and efficiency in various industries such as manufacturing, logistics, transportation, and energy [5].
The Industrial Internet of Things (IIoT) is a subset of the IoT, specifically focused on industrial applications. The IIoT offers significant advantages to companies, one of which is the automatic collection of data. By incorporating intelligent sensors into machinery and equipment, businesses can gather comprehensive data from all system components, including energy consumption and production levels. This data can then be analyzed to monitor progress over time, improve quality control, and identify and address issues proactively, ultimately leading to enhanced efficiency. The automatic data collection process also provides companies with cost and time savings. In today’s highly competitive global market, having access to accurate and real-time data is crucial for gaining a competitive edge. The IIoT offers a range of significant benefits, one of which is the compatibility and optimization of processes. When devices and equipment are connected to the Internet, factories can gain unparalleled visibility into their operations. This enhanced visibility also generates a plethora of data that can be leveraged to enhance processes and procedures. Thus, the implementation of the IIoT can facilitate the operation of smart factories with greater efficiency and reduced downtime and waste. Additionally, the heightened visibility provided by the IIoT can aid factories in meeting regulatory compliance standards with greater ease. By closely monitoring their operations, factories can ensure that they always adhere to the latest regulatory requirements. The implementation of the IIoT technology can yield substantial reductions in operating costs by automating tasks, processes, and workflows. When smart devices and machines are connected to the Internet, businesses can obtain insights into their operations and make informed decisions that can lead to cost savings. Moreover, the IIoT can facilitate the automation of manual tasks, such as material handling and data entry, which can enable businesses to free up their employees to focus on higher-value activities and decrease labor costs. In addition to cost savings and process optimization, the IIoT can also have a significant impact on workplace safety. By linking industrial equipment and sensors to the Internet, employers can gain real-time visibility into potential safety hazards. Furthermore, it can assist employers in responding quickly to emergencies by monitoring dangerous conditions and tracking the whereabouts of employees. As a result, the IIoT can improve workplace safety by providing employers with critical data and insights that enable them to take proactive measures to mitigate risks and prevent accidents. The IIoT also offers the advantage of reducing manufacturing defects. By leveraging advanced sensor technology, the IIoT can swiftly and easily detect issues in the production process. This real-time detection enables measures to be implemented to prevent the issue from recurring. This not only results in cost and time savings but also enhances safety by guaranteeing that products are of the highest quality. Additionally, the IIoT can be employed to monitor equipment performance. By detecting issues at an early stage, more severe problems can be avoided in the future, and the life of the equipment can be prolonged. Connecting machines to the IIoT allows companies to gather data about their equipment and employ it to enhance predictive maintenance processes. Furthermore, the IIoT can monitor the performance of individual parts and identify which features are most susceptible to failure. By leveraging this data, companies can develop proactive maintenance strategies to optimize equipment performance and minimize downtime. As a result, companies can save money and increase productivity by reducing unexpected equipment failures and reducing the need for reactive maintenance [6,7].
IIoT devices are capable of handling and producing a significant amount of data, despite their low cost and limited CPU capacity, storage, and memory resources. However, these devices can be prone to security vulnerabilities if proper security measures are not implemented. Such vulnerabilities can result in unexpected behavior within private networks, which may compromise the availability of services, the confidentiality of data, and the privacy of users. Therefore, the IIoT ecosystem has become a potential target for cybercriminals, and new solutions are needed to address the threats to data protection and cybersecurity [8]. In IoT ecosystems, attention must be paid to device-level vulnerabilities, insecure communication protocols, resource constraints, and non-standard security frameworks. Some precautions should be taken to prevent inadequate access, data and privacy violations. At this point, a holistic and multi-layered approach is required for the security of the IoT ecosystem. Intensive security measures should be taken at the device level, secure communication protocols should be established, advanced monitoring and intervention techniques should be determined and implemented, and standardized security measures should be provided [9].
Numerous multinational enterprises spanning diverse sectors prioritize the integration of IIoT applications to enhance operational efficiency, capitalize on emerging business prospects, and foster agility through meticulous process monitoring. Foremost among these entities are General Electric (GE), Siemens, Bosch, Schneider Electric, ABB, IBM, Amazon Web Services, and Microsoft. For instance, GE leverages the Predix platform, grounded in IIoT, to facilitate asset performance management, predictive maintenance, and operational process optimization across various domains, including energy, healthcare, aviation, and manufacturing [10]. Siemens optimizes its operational processes across diverse sectors, including energy, transportation, and others, by leveraging the MindSphere platform. This entails the collection of data and the interconnection of devices, facilitating real-time monitoring, analysis, and optimization of critical processes. Through MindSphere, Siemens enables seamless integration of industrial machinery, sensors, and data analytics, empowering businesses to make informed decisions, enhance efficiency, and drive innovation in their operations [11]. Holmer Agricultural Machinery enhances the efficiency of its service technicians by leveraging IIoT capabilities through its remote diagnosis and maintenance package called EasyHelp 4.0. This innovative solution collects data from agricultural machinery, enabling remote monitoring and diagnostics. With EasyHelp 4.0, service technicians can efficiently analyze machinery performance, identify potential issues, and perform maintenance tasks remotely. By effectively managing the maintenance process of agricultural machinery, Holmer Agricultural Machinery ensures optimal performance and minimal downtime for its customers, ultimately improving overall operational efficiency and customer satisfaction [12]. Numerous businesses, including Europe’s leading copper producer Aurubis Olen [13], the Tokuyama Cement Plant [14], Stora Enso [15], and Jujo Thermal [16], effectively utilize IIoT to manage their processes with enhanced efficiency and effectiveness. Through the integration of IIoT technologies, these companies can monitor and optimize various aspects of their operations in real-time. Whether it is enhancing production processes, improving equipment performance, or optimizing resource utilization, IIoT empowers these businesses to make data-driven decisions, streamline workflows, and achieve higher levels of productivity and operational excellence.
In the literature, IoT selection and performance evaluation have been made by different researchers using different multi-criteria decision-making (MCDM) methods [17–21]. Many developing countries and SMEs are aiming to transition to Industry 4.0 by integrating IoT service providers into their production processes. However, there is a gap in the literature regarding the evaluation criteria for selecting an IIoT service provider and how to evaluate the performance of existing providers. To address this gap, this study will seek answers to two questions. The first question is what evaluation criteria should be considered when selecting an IIoT service provider. The second question is how to assess the performance of existing service providers. To answer these questions, the Technique for Order of Preference by Similarity to Ideal Solution (TOPSIS) method, based on circular intuitionistic fuzzy sets, will be applied in an SME company operating in the automotive industry and exporting from Turkey.
In the subsequent section of the research, a thorough examination of pertinent literature utilizing the keywords “IIoT” and “TOPSIS” will be outlined. Following this, the third segment will elucidate the procedural steps of the TOPSIS approach grounded in circular intuitionistic fuzzy sets methodology, which constitutes the methodological framework employed in this investigation. Subsequently, the fourth chapter will entail the practical application of the proposed method within the context of an exporting business, accompanied by an exposition of the resultant findings. Finally, the concluding section will encompass a discussion of the research’s primary objectives, the interpretation of the obtained results, the delineation of the study’s limitations, and recommendations for future research endeavors.
In this section, a synthesis of existing literature about studies on both IoT and IIoT, as well as the TOPSIS method, will be provided.
On 19 March, 2023, a search was conducted on the Web of Science database using the keywords “Internet of Things” or “Industrial Internet of Things,” resulting in 4247 publications. The search did not include the use of abbreviations “IoT” or “IIoT” as it led to many studies that were not directly related to the topic of interest. These publications span the years between 2011 and 2023, indicating an increasing interest in the subject over time as shown in Fig. 1 [22]. The 4247 publications are classified as 2710 articles, 1380 proceeding papers, 106 review articles, 77 book chapters, and 2 books.
Figure 1: Distribution of “IoT” or “IIoT” studies by years (Reprinted with permission from reference [22]. 2023, Web of Science)
In 2023, Rajawat et al. conducted a study in which they developed a new, flexible model that can address challenges and enhance productivity. The study explored the use of “Reinforcement Learning” in industrial production processes that utilize IIoT systems. The analysis demonstrated that the proposed model showed enhancements with adaptive and dynamic decision controls, specifically in harsh industrial environments [23]. Studies about IoT or IIoT in the literature are summarized in Table 1.
In the scholarly literature, numerous studies on TOPSIS from various researchers and perspectives have been conducted. As of 21 March, 2023, a comprehensive search on the Web of Science database using the keyword “fuzzy TOPSIS” yielded 6,595 results when selecting all fields. Spanning from 1993 to 2023, this investigation examined a total of 5641 articles, 92 review articles, 927 proceeding papers, 65 book chapters, and other material types. These materials encompassed publications dating from the earliest record in 1985 to the most recent contribution in 2023. The database-indexed contents from the Web of Science served as the primary source for this examination. Fig. 2 illustrates the distribution of “fuzzy TOPSIS” studies across various years, offering valuable insights into the temporal trends and prevalence of research in this domain [33].
Figure 2: Distribution of “fuzzy TOPSIS” studies by years (Reprinted with permission from reference [33]. 2023, Web of Science)
Intuitionistic fuzzy sets are a technique that can be used to handle uncertainty in data by utilizing two membership degrees. The concept of entropy is a useful tool for characterizing the degree of uncertainty inherent in fuzzy information. In a recent study, Jin et al. [34] have proposed a novel approach to calculate entropy, which provides a new perspective on uncertainty measurement. The proposed entropy calculation method was implemented in resolving MCDM quandaries. In response to the constraints associated with conventional score functions, a novel score function for intuitionistic fuzzy numbers was introduced, grounded in a probabilistic framework. Subsequently, an entropy index was formulated leveraging this innovative score function. To streamline medical treatment selection processes, this entropy index was integrated with the three-way ranking method and TOPSIS (Technique for Order Preference by Similarity to Ideal Solution). A comprehensive summary of pertinent studies employing TOPSIS in the literature is presented in Table 2.
Fuzzy sets serve as a valuable tool in addressing risk-laden problems characterized by intense subjective evaluations [49]. This section will delve into the utilization of circular intuitionistic fuzzy sets and expound upon the operational procedures of the TOPSIS model founded on circular intuitionistic fuzzy sets, as pioneered by Kahraman et al. [50]. TOPSIS, recognized as one of the prominent multi-criteria decision-making methods, relies on Euclidean distance measurement as its foundational principle. The classical TOPSIS approach develops a solution that is close to the positive solution and far from the negative solution. In this method, precise value judgments are used. However, since decision-makers often have uncertain judgments in decision-making problems, this classical approach is inadequate for solving the problem. To overcome this deficiency, TOPSIS methods based on different fuzzy numbers are frequently used in the literature [50].
3.1 Circular Intuitionistic Fuzzy Sets (C-IFS)
Let denote the universal set, which is a non-empty and finite set as “U”. Within this framework, the intuitionistic fuzzy, IF (I) set and circular intuitionistic fuzzy, C-IF (I´) sets are defined as follows [51–54]:
In this context
For any element of
where:
The theoretical formulation provided in Eq. (6) generates five distinct circle forms, as illustrated in Fig. 3. Notably, the radius ranges from 0 and
Figure 3: The geometrical explication of an IF and a C-IF numbers (Reprinted with permission from Ref [54]. 2023, Ting-Yu Chen)
An
where
In the literature, various types of fuzzy numbers are encountered, among which Pythagorean fuzzy sets and q-rung orthopair fuzzy sets stand out as particularly significant. The theory of Intuitionistic Fuzzy Sets (IFS) was pioneered by Atanassov in 1983, leveraging conventional fuzzy sets to establish a framework for intuitive reasoning and decision-making. Circular intuitionistic fuzzy sets were also developed by Atanassov [52] and are characterized by a circle as well as membership and non-membership degrees as extensions of IFSs [53]. Circular intuitionistic fuzzy numbers are very capable of representing vague and imprecise judgments. These fuzzy sets have a circular component to represent uncertainty more flexibly and intuitively. In general, circular intuitionistic fuzzy numbers are widely used because they can deal with uncertainty, subjectivity and vague situations that arise in multi-criteria decision-making methods [54]. Additionally, C-IFNs enable the collection of multiple criteria using various aggregation operators to determine the overall utility of alternatives. C-IFNs allow for detailed potential evaluation of each alternative, considering the circularity and uncertainty of each C-IFN in this assembly process. C-IFNs are effective in solving the uncertainty and ambiguity processes encountered in the decision-making process, especially in cases where triangular, spherical, and Pythagorean fuzzy numbers may be insufficient. These numbers offer a more flexible framework than classical fuzzy numbers. Due to their circular structure, it is possible to represent non-linear relationships more accurately with these numbers. Unlike other fuzzy numbers, C-IFNs handle seasonality and cyclicality more effectively. In addition, they offer a more detailed and rich evaluation in terms of linguistic variables. Since these numbers consider situations of uncertainty and ambiguity in a broader context, the decisions made are said to be more reliable. It also has a very common application area such as engineering, health, social sciences and finance [52,54]. Given their capacity to manage cyclical relationships and uncertainty, Circular Intuitionistic Fuzzy Numbers (C-IFNs) are adeptly suited for the modeling and analysis of real-world datasets characterized by intricate patterns and uncertainties. The geometrical interpretation of numbers for both an IF set and a C-IF set is depicted in Fig. 3 [54].
C-IFs represent a robust data source that mitigates ambiguity and uncertainty. For instance, data acquired from sensors deployed on machinery within a manufacturing setting may inherently exhibit variations or inaccuracies stemming from environmental factors or other sources. C-IFs, owing to their inherent structure, effectively address this uncertainty, thereby facilitating enhanced precision in maintenance scheduling and planning. Analogously, in the healthcare domain, evaluating patient data utilizing C-IFs facilitates the derivation of more precise diagnostic assessments and treatment strategies. The adoption of these numerical constructs proves efficacious in addressing real-world challenges across diverse contexts.
3.2 TOPSIS Based on Circular Intuitionistic Fuzzy Sets (C-IFs TOPSIS)
TOPSIS is one of the multi-criteria decision-making methods used to evaluate and rank alternatives according to certain criteria. Fuzzy set theory successfully handles the uncertainty and imprecision in linguistic evaluations using fuzzy numbers. Circular heuristic fuzzy sets help experts define these degrees by considering the uncertainties in membership (belonging) and non-membership (non-belonging) degrees.
Step 1. Let determine the set of alternatives
Step 2. To construct the decision matrices consisting of linguistic terms based on Decision Makers’ (DMs) opinions using the scale provided in Table 3, each DM fills in the decision matrix using the linguistic scale. The decision matrix typically contains rows representing alternatives and columns representing criteria.
Step 3. Each decision matrix (d) created by the experts is transformed into intuitionistic fuzzy numbers (IFNs) using Table 3.
Step 4. Decision matrices created by all decision makers are aggregated by using Eq. (8) to form an intuitionistic fuzzy decision matrix.
The variable
Step 5. The radius of each IF value is calculated, and it is the maximum radius of the Euclidean distance given in Eq. (9).
Step 6. Optimistic and pessimistic decision matrices
Here
where
Step 7. Each decision maker evaluates each criterion based on the scale provided in Table 4. The weight row vector, which comprises intuitionistic fuzzy values, is denoted as
Step 8. The aggregated intuitionistic fuzzy criteria weight matrix is derived using the intuitionistic fuzzy weighted geometric (IFWG) operator. This operator combines the individual criterion weight matrices provided by each expert, as described by the following equation:
Here
Step 9. The radius values for each criterion are computed using Eq. (9). The maximum radius lengths are determined by considering the radius lengths obtained by each expert for the
Step 10. Criterion weight matrices are created depending on whether the expert is optimistic or pessimistic. The optimistic and pessimistic criterion weight matrix is formed by using Eqs. (13) and (14), respectively.
Step 11. The weighted optimistic decision matrix
Step 12. The positive ideal solution and negative ideal solution, based on the optimistic matrix, are determined using the score and accuracy functions provided in the equations below. Similarly, the positive ideal solution and negative ideal solution, based on the pessimistic matrix, are also computed using the same score and accuracy functions.
The positive ideal solution
The positive ideal solution
In this context,
Step 13. Distance measures are calculated for each alternative according to the positive and negative ideal solutions. The distance measures to the positive and negative ideal solutions based on the optimistic matrix are calculated by the following equations:
The distance measures to the positive and negative ideal solutions based on the pessimistic matrix are calculated using the following equations. The degree of hesitancy (indeterminacy) is computed as
Step 14. The relative closeness coefficient based on the optimistic and pessimistic matrices is calculated using the following equations, respectively:
Step 15. The following equation is utilized to compute the composite ratio score for ranking the alternatives. λ represents the weights assigned to the optimistic and pessimistic attitudes of the experts.
Step 16. The alternatives are ranked based on their final score. The best alternative(s) are selected in descending order of relative closeness coefficient values.
4 Evaluation of IIoT Service Providers
This section will assess the process of evaluating an IIoT service provider for the production line of a company belonging to the SME category and involved in export activities. The assessment will be conducted by a group of five experts in the field, including two academic personnel and three senior managers from the company. The evaluation will consider a range of factors to determine the most suitable IIoT service provider for the company’s needs. The proposed flowchart of the research is illustrated in Fig. 4.
Figure 4: The proposed flowchart
Upon examination of the flowchart, the initial step involves precisely and clearly defining the problem under investigation. Subsequently, a decision-making team comprising subject matter experts is assembled. Through a synthesis of expert opinions and an extensive literature review, criteria and potential alternatives pertinent to addressing the identified problem are delineated. Following this, a linguistic scale for problem resolution is established, and decision-makers are tasked with evaluating the criteria and alternatives against this scale. This evaluation yields initial decision matrices. The subsequent resolution of the problem entails employing the procedural steps of the TOPSIS method based on circular intuitive fuzzy numbers. Ultimately, the most suitable alternative is determined by systematically listing and evaluating the available options.
4.1 Determination Criteria and Alternatives
The evaluation of the IIoT service providers should consider the criteria outlined in Table 5, which are based on a thorough review of the literature and expert opinions. Following a preliminary evaluation with IoT service providers operating in the manufacturing industries, the decision-maker team reduced the number of alternatives to seven. The alternatives that successfully passed the pre-selection process are detailed in Table 6.
4.2 Application of TOPSIS Based on CIFs
At this stage, the selection process for the most appropriate Industrial Internet of Things (IIoT) service provider involves evaluating seven different alternatives. The initial step involved requesting the assessment of each alternative by five subject matter experts, who evaluated them based on nine different criteria. The initial assessment matrix obtained is presented in Table 7. Five decision-makers evaluated seven existing alternatives according to nine different evaluation criteria. During this evaluation, the decision-making team used the linguistic evaluation scale in Table 3. For example, as a result of the evaluation of each alternative according to the C1 (industry expertise) criterion by the first decision maker, A1 has the best value among the alternatives, followed by A4 and A7 alternatives.
Next, an aggregated decision matrix was generated, presented in Table 8, which utilized the decision matrices of each expert and Eq. (8). The decision matrix is comprised of intuitionistic fuzzy values.
The lengths of the radius, which are based on decision matrices produced by each expert, are calculated using Eq. (9). The circular intuitionistic fuzzy decision matrix shown in Table 9 is then created using the obtained radius lengths.
Optimistic and pessimistic matrices are obtained using Eqs. (10) and (11), respectively (Tables 10 and 11).
The experts involved in the study evaluated determined criteria by using Table 4. Based on the evaluation of the criteria, the results were then compiled into Table 12. The criteria evaluated by the experts were aggregated using Eq. (12). Here, the experience and knowledge of each expert are considered equally.
The procedures utilized to compute the optimistic and pessimistic decision matrices were similarly applied in evaluating the criteria, resulting in the derivation of the optimistic and pessimistic criteria weight matrices, as shown in Table 13.
Based on the evaluation results by experts, security was identified as the most crucial criterion, while usability was found to be the least significant. The security criteria ranked highest, followed by cost-effectiveness, data analytics, flexibility and scalability, reliability, and customer support criteria, in that order. Certain precautionary measures can be implemented by small and medium-sized enterprises (SMEs) to enhance security within their operational frameworks. One such measure involves the segmentation of their networks into smaller units, facilitated by the deployment of conventional security mechanisms including firewalls, subnets, and Virtual Local Area Networks (VLANs). Additionally, the integration of microcontrollers such as Trusted Platform Modules (TPMs) can fortify security measures by encapsulating critical components such as credentials, passwords, encryption keys, or public certificates. Access controls within SMEs can be tailored to align with specific organizational roles, qualifications, and policies, thereby ensuring stringent security protocols [65]. The adoption of blockchain technology emerges as a robust strategy to safeguard Industrial Internet of Things (IIoT) data flows [61,62]. Furthermore, augmenting access authentication processes with multifactor authentication methodologies, such as code generation, facial recognition, or biometric data in conjunction with traditional password protocols, can significantly bolster security parameters. Moreover, the establishment of secure remote access channels for employees is imperative, necessitating vigilant monitoring of network traffic for any anomalies through intrusion detection and prevention systems (IDS/IPS). Regular updates for IIoT devices and applications should be rigorously implemented to mitigate potential vulnerabilities and maintain optimal security standards [65].
By employing Eqs. (15) and (16), weighted optimistic and pessimistic decision matrices are generated, as presented in Tables 14 and 15, respectively.
The positive ideal solution
The separation measures are derived through Eqs. (23)–(26), which calculates the distances from each alternative to positive and negative ideal solutions. These solutions are based on optimistic and pessimistic matrices (Table 17).
The relative closeness coefficient based on the optimistic and pessimistic matrix is calculated using Eqs. (27) and (28) (Table 18).
The composite ratio score for ranking the alternatives is calculated by using Eq. (29) and the results are given in Table 19. The weights of the optimistic and pessimistic attitudes of the experts were accepted equally.
In this section, a sensitivity analysis is conducted to assess the reaction of the problem under varying conditions. Table 20 depicts the ranking of alternatives for different weights of the optimistic and pessimistic attitudes of the experts. Across all values of λ, the order of the first two and the last two alternatives remained unchanged. Minor variations were observed in the rankings of other alternatives for different λ values.
Each criterion was evaluated according to 9 different linguistic assessment scales from EHI to ELI and the rankings of the alternatives were tested. Nine different evaluation scales for nine different criteria resulted in 81 different results. When Fig. 5 is examined, A6 has been identified as the best IIoT service provider for several different criteria weights. A6 took place in second place for C2-EHI, C7-BAI, C7-LI, C7-VLI, C8-EHI and C9-ELI; third place for C7-ELI and fourth place for C9-EHI.
Figure 5: Ranking of alternatives based on different criteria weights
In this section, the problem that is the subject of the study will be solved with other methods used in solving multi-criteria decision-making problems and the obtained results will be examined. The problem was also solved by ARAS, COPRAS, EDAS, MOORA and CODAS methods based on triangular fuzzy numbers (TFNs) and the results are shown in Table 21.
When the table is examined, A7 was determined as the best alternative for CODAS, EDAS and MOORA methods based on TFNs, while A7 took the second place for C-IFN TOPSIS, TFN-ARAS and TFN-COPRAS methods, and A6 took the first place. Although there are some minor changes in the rankings according to the methods when Spearman’s correlation coefficient was calculated, it was 0.89 for TOPSIS with ARAS and COPRAS; for TOPSIS with CODAS, EDAS and MOORA it was 0.75. This shows that there is a positive and strong relationship between the rankings.
When solutions of different multi-criteria decision-making methods are compared, different results can be obtained. The assumptions underlying each method and the process steps of each method may differ from each other. Since different steps are involved in determining the weights of the criteria used in the evaluation of alternatives at the decision-making stage, the criterion weights take different values from each other, which changes the ranking of the alternatives. In addition, different mathematical and statistical methods are used when collecting evaluations made by more than one decision-maker. This may cause differences in the ranking of alternatives. Uncertainty is a very natural situation in solving multi-criteria decision-making problems. Depending on the solution method used, uncertainty is introduced into the problem using probabilistic models, fuzzy logic, or interval methods. This difference may cause the ranking of the alternatives to be different. For this reason, the solution of the problem will be effective by determining the methods compatible with the characteristics of the problem and the preferences of the decision maker. The robustness of the result obtained for the problem can be investigated using different methods.
The Internet of Things (IoT) is a fundamental technology of digital transformation and is considered to be at the forefront of this field. Its underlying principle is that an object equipped with sensors, which can constantly measure or detect certain situations, transmits information to a central location. This information is evaluated, and subsequent actions are based on this analysis. The primary objective of businesses, irrespective of their size, is to leverage Internet of Things (IoT) technology to equip various elements such as production lines, machines, products, semi-finished goods, and personnel with smart capabilities, thereby generating valuable data. This enables organizations to achieve greater efficacy and efficiency in managing their processes through centralized control, resulting in a more dynamic and regulated workflow.
The objective of this research is to identify the criteria that should be considered when selecting an IoT service provider, particularly for small and medium-sized enterprises (SMEs), and to determine the most suitable provider from seven available alternatives based on the company’s strategy and these criteria. The research employs a multi-criteria decision-making approach to address this typical problem, utilizing the TOPSIS method based on circular intuitionistic fuzzy sets. The study involves five decision-makers who evaluate IoT service providers and criteria, and their opinions shape the criteria weights and evaluation outcomes.
While security is the most important criterion among the criteria, this criterion is followed by cost-effectiveness, data analytics, flexibility and scalability, reliability, customer support, compatibility, and industry expertise, respectively. In the last place is the usability criterion. IIoT technology facilitates the interconnection of devices, sensors, and systems within enterprises, enabling the collection and analysis of data to enhance operational efficiency, productivity, and decision-making. However, this heightened connectivity underscores the imperative and significance of security measures. IIoT systems amass extensive datasets about production, machinery, equipment, and even personnel within business operations. Such data repositories constitute proprietary and confidential information, mandating stringent security protocols for protection. Unauthorized or unregulated access to IIoT systems utilized for monitoring and managing operational workflows represents a critical security vulnerability. Inadequate security measures may render operational processes susceptible to intrusion by malicious software, potentially resulting in disruptions and detrimental impacts on enterprises. Furthermore, security considerations should extend to IIoT systems deployed within supply chain frameworks. Vulnerabilities within this interconnected chain can exert adverse repercussions across all facets of business operations. In essence, the implementation of sensitive and rigorous security measures stands as paramount for safeguarding confidential data, ensuring the continuity of operational processes, and upholding the resilience of supply chain operations. Furthermore, amidst the pervasive global economic downturn, small and medium-sized enterprises (SMEs) are particularly apprehensive about their investment decisions. In addition, those contemplating the integration of IIoT systems cannot overlook the cost factor. Upon establishing such systems, the substantial datasets accrued from machinery, operations, and even personnel necessitate meticulous analysis and interpretation. Consequently, data analytics emerges as a pivotal criterion in this regard.
Based on the given criteria, it was concluded that the A6 alternative is the most suitable Industrial Internet of Things (IIoT) service provider, whereas the A2 IIoT service provider was found to be the least suitable. A sensitivity analysis was conducted under varying conditions and with different criterion weights, and it was found that the A6 alternative consistently performed the best, except some extreme cases.
This study aims to introduce the concept of IoT, which is a part of the digitalization processes, especially in small and medium-sized industrial enterprises and will positively affect the performance of enterprises and contribute to the decision-making processes of enterprises at this point. The biggest limitation of the study is that research was conducted for a single sector in a single country. While this study was conducted within a specific sector and country, businesses seeking to implement an IIoT system must heed these criteria, irrespective of their geographic location or industry. Considerations such as security, cost, and data analytics are likely to feature prominently in the decision-making processes of all business managers. Furthermore, IIoT service providers participating in the evaluation are entities serving clients globally. Consequently, they stand to derive insights from the findings of this study for application across diverse geographical and sectoral contexts. Multi-criteria decision-making problems are problems in which uncertainty and subjective value judgments are intense. The inability to form a homogeneous decision-maker team is a limitation.
The same problem may be solved in the future for a wider range of IIoT service providers under different evaluation criteria. Evaluation criteria and alternatives can be compared again for small and medium-sized enterprises in different sectors on a global scale. Additionally, the results can be tested in larger enterprises. Solving different MCDM methods based on different fuzzy numbers under different distance measurements can also be listed in future studies.
Acknowledgement: The author would like to thank the editors and reviewers for their valuable work, as well as my husband Burak and my son Doruk for their valuable support during the research process.
Funding Statement: The author received no specific funding for this study.
Availability of Data and Materials: The author confirms that the data supporting the findings of this study are available within the article.
Conflicts of Interest: The author declares that they have no conflicts of interest to report regarding the present study.
References
1. M. Aziez, S. Benharzallah, and H. Bennoui, “A full comparison study of service discovery approaches for internet of things,” Int. J. Pervasive Comput., vol. 15, no. 1, pp. 30–56, Jul. 2019. doi: 10.1108/IJPCC-04-2019-0038. [Google Scholar] [CrossRef]
2. P. Asghari, A. M. Rahmani, and H. H. S. Javadi, “Service composition approaches in IoT: A systematic review,” J. Netw. Comput. Appl., vol. 120, no. 4, pp. 61–77, Oct. 2018. doi: 10.1016/j.jnca.2018.07.013. [Google Scholar] [CrossRef]
3. Q. Wang, X. Zhu, Y. Ni, L. Gu, and H. Zhu, “Blockchain for the IoT and industrial IoT: A review,” Internet Things, vol. 10, no. 2, pp. 100081, Jun. 2020. doi: 10.1016/j.iot.2019.100081. [Google Scholar] [CrossRef]
4. S. Ding, A. Tukker, and H. Ward, “Opportunities and risks of internet of things (IoT) technologies for circular business models: A literature review,” J. Environ. Manag., vol. 336, no. 7084, pp. 117662, Jun. 2023. doi: 10.1016/j.jenvman.2023.117662. [Google Scholar] [PubMed] [CrossRef]
5. S. Kim, Y. Suh, and H. Lee, “What IoT devices and applications should be connected? Predicting user behaviors of IoT services with node2vec embedding,” Inf. Process. Manag., vol. 59, no. 2, pp. 102869, Mar. 2022. doi: 10.1016/j.ipm.2022.102869. [Google Scholar] [CrossRef]
6. C. Lamprecht, H. Gebauer, E. Fleisch, and F. Wortmann, “A KPI set for steering the IoT business in product companies,” Res. Technol. Manag., vol. 65, no. 2, pp. 53–63, Feb. 2022. doi: 10.1080/08956308.2022.2015951. [Google Scholar] [CrossRef]
7. Litum, “The benefits of industrial IoT to businesses,” Accessed: Jan. 1, 2023. [Online]. Available: https://litum.com/the-benefits-of-industrial-iot-businesses [Google Scholar]
8. N. Prazeres, R. L. D. C. Costa, L. Santos, and C. Rabadao, “Engineering the application of machine learning in an IDS based on IOT traffic flow,” Intell. Syst. Appl., vol. 17, pp. 200189, Feb. 2023. doi: 10.1016/j.iswa.2023.200189. [Google Scholar] [CrossRef]
9. K. Potter, J. Oloyede, and F. Olaoye, “Securing the internet of things (IoT) ecosystem: Challenges and solutions in cybersecurity,” J. Internet Things, Jan. 2024. Accessed: May 17, 2024. [Online]. Available: https://www.researchgate.net/publication/377207519_Securing_the_Internet_of_Things_IoT_Ecosystem_Callenges_and_Solutions_in_Cybersecurity [Google Scholar]
10. C. Parris, “What is the industrial internet of things (IIoT)?” Accessed: May 17, 2024. [Online]. Available: https://www.ge.com/digital/blog/what-industrial-internet-things-iiot [Google Scholar]
11. Siemens, “IIoT evolution: An approach to reuse and scale your IIoT technology investment,” Accessed: May 17, 2024. [Online]. Available: https://resources.sw.siemens.com/en-US/white-paper-iiot-evolution-reuse-and-scale-your-iiot-technology [Google Scholar]
12. Bosch, “HOLMER: How to make the most of agricultural IoT data,” Accessed: May 17, 2024. [Online]. Available: https://bosch-iot-suite.com/knowledge-center/customer-reference/holmer-how-to-make-the-most-of-agricultural-iot-data/ [Google Scholar]
13. ABB, “Aurubis Olen seeks a remedy for an unexpected downtime,” Accessed: May 17, 2024. [Online]. Available: https://stories.ability.abb.com/aurubis-olen/situation.html [Google Scholar]
14. ABB, “Tokuyama cement plant uses ABB industrial software to reduce energy consumption,” Accessed: May 17, 2024. [Online]. Available: https://stories.ability.abb.com/tokuyama/?_ga=2.189943656.236566383.1715951206-1056602989.1715951206 [Google Scholar]
15. ABB, “Renewble packaging producer seeks to improve productivity,” Accessed: May 17, 2024. [Online]. Available: https://stories.ability.abb.com/stora-enso/situation.html [Google Scholar]
16. ABB, “Jujo thermal seeks to upgrade its manufacturing business systems,” Accessed: May 17, 2024. [Online]. Available: https://stories.ability.abb.com/jujo-thermal/situation.html [Google Scholar]
17. Y. Badulescu, M. K. Tiwari, and N. Cheikhrouhou, “MCDM approach to select IoT devices for the reverse logistics process in the clinical trials supply chain,” Proc. 10th IFAC Conf. Manuf. Model., Manag. Control, Nanttes, Frances, vol. 55, no. 10, pp. 43–48, Jun. 2022. doi: 10.1016/j.ifacol.2022.09.365. [Google Scholar] [CrossRef]
18. B. Alojaiman, “A multi-criteria decision-making process for the selection of an efficient and reliable IoT application,” Processes, vol. 11, no. 5, pp. 1313, Apr. 2023. doi: 10.3390/pr11051313. [Google Scholar] [CrossRef]
19. M. Lin, C. Huang, Z. Xu, and R. Chen, “Evaluating IoT platforms using integrated probabilistic linguistic MCDM method,” IEEE Internet Things J., vol. 7, no. 11, pp. 11195–11208, May 2020. doi: 10.1109/JIOT.2020.2997133. [Google Scholar] [CrossRef]
20. G. Illieva and T. Yankova, “IoT system selection as a fuzzy multi-criteria problem,” Sensors, vol. 22, no. 11, pp. 4110, Jun. 2022. doi: 10.3390/s22114110. [Google Scholar] [PubMed] [CrossRef]
21. M. H. Kadhim and F. Mardukhi, “A novel IoT application recommendation system using metaheuristic multi-criteria analysis,” Comput. Syst. Sci. Eng., Oct. 2020. doi: 10.32604/csse.2021.014608. [Google Scholar] [CrossRef]
22. Web of Science, Accessed: Mar. 19, 2023. [Online]. Available: https://www.webofscience.com/wos/woscc/summary/d7a46765-1307-4c36-a172-bea638a27a37-ea33a88b/relevance/1 [Google Scholar]
23. A. S. Rajawat, S. B. Goyal, C. Chauhan, P. Bedi, M. Prasad and T. Jan, “Cognitive adaptive systems for industrial internet of things using reinforcement algorithm,” Electronics, vol. 12, no. 1, pp. 217, Jan. 2023. doi: 10.3390/electronics12010217. [Google Scholar] [CrossRef]
24. N. Siegfried, T. Rosenthal, and A. Benlian, “Blockchain and the industrial internet of things a requirement taxonomy and systematic fit analysis,” J. Enterp. Inf. Manage., vol. 35, no. 6, pp. 1454–1476, Jan. 2020. doi: 10.1108/JEIM-06-2018-0140. [Google Scholar] [CrossRef]
25. H. Sarjan, A. Ameli, and M. Ghafouri, “Cyber-security of industrial internet of things in electric power systems,” IEEE Access, vol. 10, no. 1, pp. 92390–92409, Aug. 2022. doi: 10.1109/ACCESS.2022.3202914. [Google Scholar] [CrossRef]
26. G. Zhang, C. H. Chen, X. G. Cao, R. Y. Zhong, X. Y. Duan and P. F. Li, “Industrial internet of things-enabled monitoring and maintenance mechanism for fully mechanized mining equipment,” Adv. Eng. Inform., vol. 54, no. 5, pp. 101782, Oct. 2022. doi: 10.1016/j.aei.2022.101782. [Google Scholar] [CrossRef]
27. M. Alabadi, A. Habbal, and X. Wei, “Industrial internet of things: Requirements, architecture, challenges, and future research directions,” IEEE Access, vol. 10, no. 1, pp. 66374–66400, Jun. 2022. doi: 10.1109/ACCESS.2022.3185049. [Google Scholar] [CrossRef]
28. L. Haghnegahdar, S. S. Joshi, and N. B. Dahotre, “From IoT-based cloud manufacturing approach to intelligent additive manufacturing: Industrial internet of things-an overview,” Int. J. Adv. Manufact. Tech., vol. 119, no. 3–4, pp. 1461–1478, Mar. 2022. doi: 10.1007/s00170-021-08436-x. [Google Scholar] [CrossRef]
29. A. Krommuang and O. Suwunnamek, “Internet of things (IoT) application for management in automotive parts manufacturing,” Int. J. Adv. Comput. Sc. Appl., vol. 13, no. 4, pp. 639–650, 2022. doi: 10.14569/issn.2156-5570. [Google Scholar] [CrossRef]
30. D. H. Shih, T. W. Wu, M. H. Shih, G. W. Chen, and D. C. Yen, “Hyperledger fabric access control for industrial internet of things,” Appl. Sci., vol. 12, no. 6, pp. 3125, Mar. 2022. doi: 10.3390/app12063125. [Google Scholar] [CrossRef]
31. R. L. Kumar, F. Khan, S. Kadry, and S. Rho, “A survey on blockchain for industrial internet of things,” Alex. Eng. J., vol. 61, no. 8, pp. 6001–6022, Aug. 2022. doi: 10.1016/j.aej.2021.11.023. [Google Scholar] [CrossRef]
32. Q. Qi, Z. Xu, and P. Rani, “Big data analytics challenges to implementing the intelligent industrial internet of things (IIoT) systems in sustainable manufacturing operations,” Technol. Forecast. Soc., vol. 190, no. 2, pp. 122401, May 2023. doi: 10.1016/j.techfore.2023.122401. [Google Scholar] [CrossRef]
33. Web of Science. Accessed: Mar. 19, 2023. [Online]. Available: https://www.webofscience.com/wos/woscc/summary/4d8688b5-38de-4a24-ab5f-027885176e86-ea338827/relevance/1 [Google Scholar]
34. J. Jin and H. Garg, “Intuitionistic fuzzy three way ranking based TOPSIS approach with a novel entropy measure and its application to medical treatment selection,” Adv. Eng. Softw., vol. 180, pp. 103459, Jun. 2023. doi: 10.1016/j.advengsoft.2023.103459. [Google Scholar] [CrossRef]
35. T. Umamakeswari, “Evaluating the quality factors of leaf plates by fuzzy TOPSIS method,” Mater. Today Proc., vol. 80, no. 2, pp. 1562–1566, 2023. doi: 10.1016/j.matpr.2023.01.390. [Google Scholar] [CrossRef]
36. V. Singh, V. Kumar, and V. B. Singh, “A hybrid novel fuzzy AHP-TOPSIS technique for selecting parameter-influencing testing in software development,” Decis. Anal. J., vol. 6, no. 1, pp. 100159, Mar. 2023. doi: 10.1016/j.dajour.2022.100159. [Google Scholar] [CrossRef]
37. S. S. Lin, A. Zhou, and S. L. Shen, “Safety assessment of excavation system via TOPSIS-based MCDM modelling in fuzzy environment,” Appl. Soft Comput., vol. 138, no. 2, pp. 110206, Mar. 2023. doi: 10.1016/j.asoc.2023.110206. [Google Scholar] [CrossRef]
38. P. Bhatio and N. Diaz-Elsayed, “Facilitating decision-making for the adoption of smart manufacturing technologies by SMEs via fuzzy TOPSIS,” Int. J. Prod. Econ., vol. 257, no. 8, pp. 108762, Mar. 2023. doi: 10.1016/j.ijpe.2022.108762. [Google Scholar] [CrossRef]
39. L. T. H. Lan, D. T. T. Hien, N. T. Thong, and F. Smarandache, “An ANP-TOPSIS model for tourist destination choice problems under temporal neutrosophic environment,” Appl. Soft Comput., vol. 136, no. 7, pp. 110146, Mar. 2023. doi: 10.1016/j.asoc.2023.110146. [Google Scholar] [CrossRef]
40. Z. Huang, C. Yang, X. Zhou, and W. Gui, “An improved TOPSIS-based multi-criteria decision-making approach for evaluating the working conditions of the aluminium cell,” Eng Appl. Artif. Intell., vol. 117, no. 5, pp. 105599, Jan. 2023. doi: 10.1016/j.engappai.2022.105599. [Google Scholar] [CrossRef]
41. H. Kaur, S. Gupta, and A. Dhingra, “Selection of solar panel using entropy-TOPSIS technique,” in Today Proc., Feb. 2023. doi: 10.1016/j.matpr.2023.02.034. [Google Scholar] [CrossRef]
42. M. Sarwar, G. Ali, and N. R. Chaudhry, “Decision-making model for failure modes and effect analysis based on rough fuzzy integrated clouds,” Appl. Soft Comput., vol. 136, no. 3, pp. 110148, Mar. 2023. doi: 10.1016/j.asoc.2023.110148. [Google Scholar] [CrossRef]
43. Y. An, X. Tan, B. Gu, K. Zhu, L. Shi and Z. Ding, “An assessment of renewable energy development in belt and road initiative countries: An entropy and TOPSIS approach,” Energy Rep., vol. 9, pp. 3545–3560, Nov. 2023. doi: 10.1016/j.egyr.2023.01.129. [Google Scholar] [CrossRef]
44. R. Mohamed Mouhoumed, Ö. Ekmekcioğlu, and M. Özger, “A hybrid MCDA approach for delineating sites suitable for artificial groundwater recharge using drywells,” J. Hydrol., vol. 620, pp. 129387, May 2023. doi: 10.1016/j.jhydrol.2023.129387. [Google Scholar] [CrossRef]
45. Y. C. Chen, P. A. Chateau, and Y. C. Chang, “Hybrid multiple-criteria decision-making for bulk carriers ballast water management system selection,” Ocean Coast. Manag., vol. 234, no. 3, pp. 106456, Mar. 2023. doi: 10.1016/j.ocecoaman.2022.106456. [Google Scholar] [CrossRef]
46. J. L. Miguez, E. R. Lopez, J. Porteiro, and R. Perez-Orozco, “Selection of non-financial sustainability indicator as key elements for multi-criteria analysis of hotel chains,” Sustain. Prod. Consump., vol. 35, no. 13, pp. 495–508, Jan. 2023. doi: 10.1016/j.spc.2022.12.004. [Google Scholar] [CrossRef]
47. S. Eraslan and F. Karaaslan, “A group decision making method based on TOPSIS under fuzzy soft environment,” J. New Theory, vol. 3, pp. 30–40, 2015. [Google Scholar]
48. S. Enginoğlu, S. Memiş, and F. Karaaslan, “A new approach to group decision-making method based on TOPSIS under fuzzy soft environment,” J. New Result Sci. 2019, vol. 8, no. 2, pp. 42–52, Dec. 2019. [Google Scholar]
49. X. Chen, S. Liu, R. W. Liu, H. Wu, B. Han and J. Zhao, “Quantifying Arctic oil spilling event risk by integrating an analytic network process and a fuzzy comprehensive evaluation model,” Ocean Coast. Manag., vol. 228, no. 3, pp. 106326, Sep. 2022. doi: 10.1016/j.ocecoaman.2022.106326. [Google Scholar] [CrossRef]
50. C. Kahraman and N. Alkan, “Circular intuitionistic fuzzy TOPSIS method with vague membership functions: Supplier selection application context,” Notes Int. Fuzz. Sets, vol. 27, no. 1, pp. 24–52, Jun. 2021. doi: 10.7546/nifs.2021.27.1.24-52. [Google Scholar] [CrossRef]
51. M. J. Khan, W. Kumam, and N. A. Alreshidi, “Divergence measures for circular intuitionistic fuzzy sets and their applications,” Eng. Appl. Artif. Intell., vol. 116, no. 7, pp. 105455, Nov. 2022. doi: 10.1016/j.engappai.2022.105455. [Google Scholar] [CrossRef]
52. K. T. Atanassov, “Circular intuitionistic fuzzy sets,” J. Amb. Intell. Smart En, vol. 39, no. 5, pp. 5981–5986, 2020. doi: 10.3233/JIFS-189072. [Google Scholar] [CrossRef]
53. K. Atanassov and E. Marinov, “Four distances for circular intuitionistic fuzzy sets,” Mathematics, vol. 9, no. 10, pp. 1121, May 2021. doi: 10.3390/math9101121. [Google Scholar] [CrossRef]
54. T. Y. Chen, “A circular intuitionistic fuzzy evaluation method based on distances from the average solution to support multiple criteria intelligent decisions involving uncertainty,” Eng. Appl. Artif. Intell., vol. 117, pp. 105499, Jan. 2023. doi: 10.1016/j.engappai.2022.105499. [Google Scholar] [CrossRef]
55. B. Uslu, T. Eren, Ş. Gür, and E. Özcan, “Evaluation of the difficulties in the internet of things (IoT) with multi-criteria decision-making,” Processes, vol. 7, no. 3, pp. 164, Mar. 2019. doi: 10.3390/pr7030164. [Google Scholar] [CrossRef]
56. A. Alelaiwi, “Evaluating distributed IoT databases for edge/cloud platforms using the analytic hierarchy process,” J. Parallel Distr. Comput., vol. 124, no. 9, pp. 41–46, Feb. 2019. doi: 10.1016/j.jpdc.2018.10.008. [Google Scholar] [CrossRef]
57. S. K. Garg, S. Versteeg, and R. Buyya, “A framework for ranking of cloud computing services,” Future Gener. Comput. Syst., vol. 29, no. 4, pp. 1012–1023, Jun. 2013. doi: 10.1016/j.future.2012.06.006. [Google Scholar] [CrossRef]
58. S. Soltani, P. Martin, and K. Elgazzar, “A hybrid approach to automatic IaaS service selection,” J. Cloud Comput., vol. 7, no. 1, pp. 1–18, Jul. 2018. doi: 10.1186/s13677-018-0113-8. [Google Scholar] [CrossRef]
59. Y. Kondratenko, G. Kondratenko, and I. Sidenko, “Multi-criteria decision making for selecting a rational IoT platform,” in 2018 IEEE 9th Int. Conf. Dependable Sys., Serv., Tech. (DESSERT), Kyiv, Ukraine, May 2018, pp. 147–152. [Google Scholar]
60. C. Singla, N. Mahajan, S. Kaushal, A. Verma, and A. K. Sangaiah, “Modelling and analysis of multi-objective service selection scheme in IoT-cloud environment,” in Cognitive Computing for Big Data Systems over IoT: Frameworks, Tools and Applications, Springer Cham, Nature, Switzerland, 2018, pp. 63–77. [Google Scholar]
61. A. Yazdinejad, A. Dehghantanha, R. M. Parizi, M. Hammoudeh, H. Karimipour, and G. Srivastava, “Block hunter: Federated learning for cyber threat hunting in blockchain-based IIoT networks,” IEEE Trans. Ind. Inform., vol. 18, no. 11, pp. 8356–8366, Nov. 2022. doi: 10.1109/TII.2022.3168011. [Google Scholar] [CrossRef]
62. A. Yazdinejad, A. Dehghantanha, R. M. Parizi, G. Srivastava, and H. Karimipour, “Secure intelligent fuzzy blockchain framework: Effective threat detection in IoT networks,” Comput. Ind., vol. 144, no. 4, pp. 103801, 2023. doi: 10.1016/j.compind.2022.103801. [Google Scholar] [CrossRef]
63. E. M. Silva, C. Agostinho, and R. Jardim-Goncalves, “A multi-criteria decision model for the selection of a more suitable Internet-of-Things device,” in 2017 Int. Conf. on Eng., Technol. Innov. (ICE/ITMC), Madeira, Portugal, Jun. 2017, pp. 1268–1276. [Google Scholar]
64. E. M. Silva and R. Jardim-Gonçalves, “Multi-criteria analysis and decision methodology for the selection of internet-of-things hardware platforms,” in Technol. Innov. Smart Syst.: 8th IFIP WG 5.5/SOCOLNET Adv. Doctor. Conf. Comput., Electr. Ind. Syst., DoCEIS 2017, Costa de Caparica, Portugal, May 3–5, 2017, pp. 111–121. [Google Scholar]
65. M. Pliats, “Industrial IoT security: Top concerns and actionable strategies,” Accessed: May 6, 2024. [Online]. Available: https://www.itransition.com/iot/industrial-security [Google Scholar]
Cite This Article
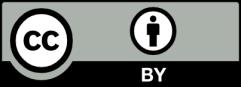