Open Access
ARTICLE
Ensemble Approach Combining Deep Residual Networks and BiGRU with Attention Mechanism for Classification of Heart Arrhythmias
1 Department of Information Systems, Al-Farabi Kazakh National University, Almaty, 050040, Kazakhstan
2 Department of Mathematical and Computer Modeling, International Information Technology University, Almaty, 050040, Kazakhstan
3 Department of Software Engineering, Satbayev University, Almaty, 050013, Kazakhstan
* Corresponding Authors: Batyrkhan Omarov. Email: ,
Computers, Materials & Continua 2024, 80(1), 341-359. https://doi.org/10.32604/cmc.2024.052437
Received 07 April 2024; Accepted 03 June 2024; Issue published 18 July 2024
Abstract
This research introduces an innovative ensemble approach, combining Deep Residual Networks (ResNets) and Bidirectional Gated Recurrent Units (BiGRU), augmented with an Attention Mechanism, for the classification of heart arrhythmias. The escalating prevalence of cardiovascular diseases necessitates advanced diagnostic tools to enhance accuracy and efficiency. The model leverages the deep hierarchical feature extraction capabilities of ResNets, which are adept at identifying intricate patterns within electrocardiogram (ECG) data, while BiGRU layers capture the temporal dynamics essential for understanding the sequential nature of ECG signals. The integration of an Attention Mechanism refines the model’s focus on critical segments of ECG data, ensuring a nuanced analysis that highlights the most informative features for arrhythmia classification. Evaluated on a comprehensive dataset of 12-lead ECG recordings, our ensemble model demonstrates superior performance in distinguishing between various types of arrhythmias, with an accuracy of 98.4%, a precision of 98.1%, a recall of 98%, and an F-score of 98%. This novel combination of convolutional and recurrent neural networks, supplemented by attention-driven mechanisms, advances automated ECG analysis, contributing significantly to healthcare’s machine learning applications and presenting a step forward in developing non-invasive, efficient, and reliable tools for early diagnosis and management of heart diseases.Keywords
Cite This Article
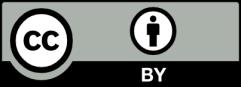
This work is licensed under a Creative Commons Attribution 4.0 International License , which permits unrestricted use, distribution, and reproduction in any medium, provided the original work is properly cited.