Open Access
ARTICLE
YOLO-O2E: A Variant YOLO Model for Anomalous Rail Fastening Detection
1 Department of Computer Science and Engineering, Chongqing University of Technology, Chongqing, 400054, China
2 Institute of Flexible Electronics (Future Technologies), Xiamen University, Xiamen, 361000, China
3 College of Mechanical Engineering, Chongqing University of Technology, Chongqing, 400054, China
* Corresponding Author: Jianxun Zhang. Email:
(This article belongs to the Special Issue: The Latest Deep Learning Architectures for Artificial Intelligence Applications)
Computers, Materials & Continua 2024, 80(1), 1143-1161. https://doi.org/10.32604/cmc.2024.052269
Received 28 March 2024; Accepted 31 May 2024; Issue published 18 July 2024
Abstract
Rail fasteners are a crucial component of the railway transportation safety system. These fasteners, distinguished by their high length-to-width ratio, frequently encounter elevated failure rates, necessitating manual inspection and maintenance. Manual inspection not only consumes time but also poses the risk of potential oversights. With the advancement of deep learning technology in rail fasteners, challenges such as the complex background of rail fasteners and the similarity in their states are addressed. We have proposed an efficient and high-precision rail fastener detection algorithm, named YOLO-O2E (you only look once-O2E). Firstly, we propose the EFOV (Enhanced Field of View) structure, aiming to adjust the effective receptive field size of convolutional kernels to enhance insensitivity to small spatial variations. Additionally, The OD_MP (ODConv and MP_2) and EMA (Efficient Multi-Scale Attention) modules mentioned in the algorithm can acquire a wider spectrum of contextual information, enhancing the model’s ability to recognize and locate objectives. Additionally, we collected and prepared the GKA dataset, sourced from real train tracks. Through testing on the GKA dataset and the publicly available NUE-DET dataset, our method outperforms general-purpose object detection algorithms. On the GKA dataset, our model achieved a mAP@0.5 value of 97.6% and a mAP@0.5:0.95 value of 83.9%, demonstrating excellent inference speed. YOLO-O2E is an algorithm for detecting anomalies in railway fasteners that is applicable in practical industrial settings, addressing the industry gap in rail fastener detection.Keywords
Cite This Article
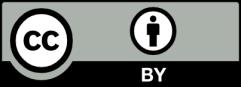