Open Access
ARTICLE
Transformer-Based Cloud Detection Method for High-Resolution Remote Sensing Imagery
1 Key Laboratory of GIS Application Research, School of Geography and Tourism, Chongqing Normal University, Chongqing, 401331, China
2 College of Computer and Information Science, Chongqing Normal University, Chongqing, 401331, China
3 Department of Military Installations, Army Logistics Academy, Chongqing, 401331, China
* Corresponding Author: Song Sun. Email:
(This article belongs to the Special Issue: The Latest Deep Learning Architectures for Artificial Intelligence Applications)
Computers, Materials & Continua 2024, 80(1), 661-678. https://doi.org/10.32604/cmc.2024.052208
Received 26 March 2024; Accepted 22 May 2024; Issue published 18 July 2024
Abstract
Cloud detection from satellite and drone imagery is crucial for applications such as weather forecasting and environmental monitoring. Addressing the limitations of conventional convolutional neural networks, we propose an innovative transformer-based method. This method leverages transformers, which are adept at processing data sequences, to enhance cloud detection accuracy. Additionally, we introduce a Cyclic Refinement Architecture that improves the resolution and quality of feature extraction, thereby aiding in the retention of critical details often lost during cloud detection. Our extensive experimental validation shows that our approach significantly outperforms established models, excelling in high-resolution feature extraction and precise cloud segmentation. By integrating Positional Visual Transformers (PVT) with this architecture, our method advances high-resolution feature delineation and segmentation accuracy. Ultimately, our research offers a novel perspective for surmounting traditional challenges in cloud detection and contributes to the advancement of precise and dependable image analysis across various domains.Keywords
Cite This Article
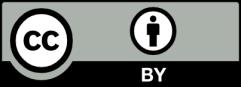
This work is licensed under a Creative Commons Attribution 4.0 International License , which permits unrestricted use, distribution, and reproduction in any medium, provided the original work is properly cited.