Open Access
ARTICLE
An Enhanced GAN for Image Generation
1 PAMI Research Group, University of Macau, Macau, 999078, China
2 School of Software, Northwestern Polytechnical University, Xi’an, 710129, China
3 Yangtze River Delta Research Institute, Northwestern Polytechnical University, Taicang, 215400, China
4 Research & Development Institute, Northwestern Polytechnical University, Shenzhen, 518057, China
* Corresponding Author: Bob Zhang. Email:
(This article belongs to the Special Issue: Multimodal Learning in Image Processing)
Computers, Materials & Continua 2024, 80(1), 105-118. https://doi.org/10.32604/cmc.2024.052097
Received 22 March 2024; Accepted 20 June 2024; Issue published 18 July 2024
Abstract
Generative adversarial networks (GANs) with gaming abilities have been widely applied in image generation. However, gamistic generators and discriminators may reduce the robustness of the obtained GANs in image generation under varying scenes. Enhancing the relation of hierarchical information in a generation network and enlarging differences of different network architectures can facilitate more structural information to improve the generation effect for image generation. In this paper, we propose an enhanced GAN via improving a generator for image generation (EIGGAN). EIGGAN applies a spatial attention to a generator to extract salient information to enhance the truthfulness of the generated images. Taking into relation the context account, parallel residual operations are fused into a generation network to extract more structural information from the different layers. Finally, a mixed loss function in a GAN is exploited to make a tradeoff between speed and accuracy to generate more realistic images. Experimental results show that the proposed method is superior to popular methods, i.e., Wasserstein GAN with gradient penalty (WGAN-GP) in terms of many indexes, i.e., Frechet Inception Distance, Learned Perceptual Image Patch Similarity, Multi-Scale Structural Similarity Index Measure, Kernel Inception Distance, Number of Statistically-Different Bins, Inception Score and some visual images for image generation.Keywords
Cite This Article
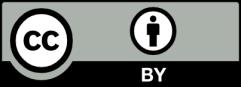
This work is licensed under a Creative Commons Attribution 4.0 International License , which permits unrestricted use, distribution, and reproduction in any medium, provided the original work is properly cited.