Open Access
ARTICLE
YOLO-Based Damage Detection with StyleGAN3 Data Augmentation for Parcel Information-Recognition System
1 Department of Electrical and Computer Engineering, Sungkyunkwan University, Suwon, 16419, Republic of Korea
2 Logistics System Research Division, Korea Railroad Research Institute, Uiwang, 16105, Republic of Korea
* Corresponding Author: Sang-Duck Lee. Email:
Computers, Materials & Continua 2024, 80(1), 195-215. https://doi.org/10.32604/cmc.2024.052070
Received 21 March 2024; Accepted 11 June 2024; Issue published 18 July 2024
Abstract
Damage to parcels reduces customer satisfaction with delivery services and increases return-logistics costs. This can be prevented by detecting and addressing the damage before the parcels reach the customer. Consequently, various studies have been conducted on deep learning techniques related to the detection of parcel damage. This study proposes a deep learning-based damage detection method for various types of parcels. The method is intended to be part of a parcel information-recognition system that identifies the volume and shipping information of parcels, and determines whether they are damaged; this method is intended for use in the actual parcel-transportation process. For this purpose, 1) the study acquired image data in an environment simulating the actual parcel-transportation process, and 2) the training dataset was expanded based on StyleGAN3 with adaptive discriminator augmentation. Additionally, 3) a preliminary distinction was made between the appearance of parcels and their damage status to enhance the performance of the parcel damage detection model and analyze the causes of parcel damage. Finally, using the dataset constructed based on the proposed method, a damage type detection model was trained, and its mean average precision was confirmed. This model can improve customer satisfaction and reduce return costs for parcel delivery companies.Keywords
Cite This Article
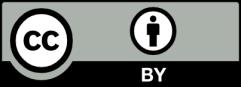