Open Access
ARTICLE
Network Security Enhanced with Deep Neural Network-Based Intrusion Detection System
1 Information Systems Department, College of Computer and Information Sciences, Princess Nourah bint Abdulrahman University, Riyadh, 11671, Saudi Arabia
2 College of Computer and Information Sciences, King Saud University, Riyadh, 11362, Saudi Arabia
3 College of Computer and Information Sciences, Prince Sultan University, Riyadh, 11586, Saudi Arabia
4 Department of Educational Technologies, College of Education, Imam Abdulrahman Bin Faisal University, P.O. Box 1982, Dammam, 31441, Saudi Arabia
* Corresponding Author: Syed Umar Amin. Email:
Computers, Materials & Continua 2024, 80(1), 1457-1490. https://doi.org/10.32604/cmc.2024.051996
Received 20 March 2024; Accepted 05 June 2024; Issue published 18 July 2024
Abstract
This study describes improving network security by implementing and assessing an intrusion detection system (IDS) based on deep neural networks (DNNs). The paper investigates contemporary technical ways for enhancing intrusion detection performance, given the vital relevance of safeguarding computer networks against harmful activity. The DNN-based IDS is trained and validated by the model using the NSL-KDD dataset, a popular benchmark for IDS research. The model performs well in both the training and validation stages, with 91.30% training accuracy and 94.38% validation accuracy. Thus, the model shows good learning and generalization capabilities with minor losses of 0.22 in training and 0.1553 in validation. Furthermore, for both macro and micro averages across class 0 (normal) and class 1 (anomalous) data, the study evaluates the model using a variety of assessment measures, such as accuracy scores, precision, recall, and F1 scores. The macro-average recall is 0.9422, the macro-average precision is 0.9482, and the accuracy scores are 0.942. Furthermore, macro-averaged F1 scores of 0.9245 for class 1 and 0.9434 for class 0 demonstrate the model’s ability to precisely identify anomalies precisely. The research also highlights how real-time threat monitoring and enhanced resistance against new online attacks may be achieved by DNN-based intrusion detection systems, which can significantly improve network security. The study underscores the critical function of DNN-based IDS in contemporary cybersecurity procedures by setting the foundation for further developments in this field. Upcoming research aims to enhance intrusion detection systems by examining cooperative learning techniques and integrating up-to-date threat knowledge.Keywords
Cite This Article
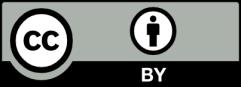
This work is licensed under a Creative Commons Attribution 4.0 International License , which permits unrestricted use, distribution, and reproduction in any medium, provided the original work is properly cited.