Open Access
ARTICLE
A GAN-EfficientNet-Based Traceability Method for Malicious Code Variant Families
School of Computer and Control Engineering, Northeast Forestry University, Harbin, 150040, China
* Corresponding Author: Li Li. Email:
Computers, Materials & Continua 2024, 80(1), 801-818. https://doi.org/10.32604/cmc.2024.051916
Received 18 March 2024; Accepted 17 May 2024; Issue published 18 July 2024
Abstract
Due to the diversity and unpredictability of changes in malicious code, studying the traceability of variant families remains challenging. In this paper, we propose a GAN-EfficientNetV2-based method for tracing families of malicious code variants. This method leverages the similarity in layouts and textures between images of malicious code variants from the same source and their original family of malicious code images. The method includes a lightweight classifier and a simulator. The classifier utilizes the enhanced EfficientNetV2 to categorize malicious code images and can be easily deployed on mobile, embedded, and other devices. The simulator utilizes an enhanced generative adversarial network to simulate different variants of malicious code and generates datasets to validate the model’s performance. This process helps identify model vulnerabilities and security risks, facilitating model enhancement and development. The classifier achieves 98.61% and 97.59% accuracy on the MMCC dataset and Malevis dataset, respectively. The simulator’s generated image of malicious code variants has an FID value of 155.44 and an IS value of 1.72 ± 0.42. The classifier’s accuracy for tracing the family of malicious code variants is as high as 90.29%, surpassing that of mainstream neural network models. This meets the current demand for high generalization and anti-obfuscation abilities in malicious code classification models due to the rapid evolution of malicious code.Keywords
Cite This Article
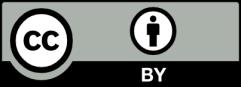
This work is licensed under a Creative Commons Attribution 4.0 International License , which permits unrestricted use, distribution, and reproduction in any medium, provided the original work is properly cited.