Open Access
ARTICLE
Novel Fractal-Based Features for Low-Power Appliances in Non-Intrusive Load Monitoring
1 Department of Electrical Engineering, University of Engineering and Technology (UET), Lahore, 54890, Pakistan
2 Department of Electrical Engineering, Government College University, Faisalabad, 38000, Pakistan
3 Department of Electrical Engineering and Technology, Muhammad Nawaz Sharif University of Engineering & Technology (MNS UET), Multan, 60000, Pakistan
* Corresponding Author: Anam Mughees. Email:
Computers, Materials & Continua 2024, 80(1), 507-526. https://doi.org/10.32604/cmc.2024.051820
Received 16 March 2024; Accepted 11 May 2024; Issue published 18 July 2024
Abstract
Non-intrusive load monitoring is a method that disaggregates the overall energy consumption of a building to estimate the electric power usage and operating status of each appliance individually. Prior studies have mostly concentrated on the identification of high-power appliances like HVAC systems while overlooking the existence of low-power appliances. Low-power consumer appliances have comparable power consumption patterns, which can complicate the detection task and can be mistaken as noise. This research tackles the problem of classification of low-power appliances and uses turn-on current transients to extract novel features and develop unique appliance signatures. A hybrid feature extraction method based on mono-fractal and multi-fractal analysis is proposed for identifying low-power appliances. Fractal dimension, Hurst exponent, multifractal spectrum and the Hölder exponents of switching current transient signals are extracted to develop various ‘turn-on’ appliance signatures for classification. Four classifiers, i.e., deep neural network, support vector machine, decision trees, and K-nearest neighbours have been optimized using Bayesian optimization and trained using the extracted features. The simulated results showed that the proposed method consistently outperforms state-of-the-art feature extraction methods across all optimized classifiers, achieving an accuracy of up to 96 % in classifying low-power appliances.Keywords
Cite This Article
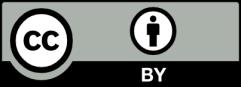