Open Access
ARTICLE
Evolutionary Variational YOLOv8 Network for Fault Detection in Wind Turbines
1 College of Information, Shenyang Institute of Engineering, Shenyang, 110136, China
2 Graduate School, Shenyang Institute of Engineering, Shenyang, 110136, China
3 College of Software, Northeastern University, Shenyang, 110169, China
* Corresponding Authors: Qingze Shen. Email: ; Tian Zhang. Email:
(This article belongs to the Special Issue: Neural Architecture Search: Optimization, Efficiency and Application)
Computers, Materials & Continua 2024, 80(1), 625-642. https://doi.org/10.32604/cmc.2024.051757
Received 14 March 2024; Accepted 16 May 2024; Issue published 18 July 2024
Abstract
Deep learning has emerged in many practical applications, such as image classification, fault diagnosis, and object detection. More recently, convolutional neural networks (CNNs), representative models of deep learning, have been used to solve fault detection. However, the current design of CNNs for fault detection of wind turbine blades is highly dependent on domain knowledge and requires a large amount of trial and error. For this reason, an evolutionary YOLOv8 network has been developed to automatically find the network architecture for wind turbine blade-based fault detection. YOLOv8 is a CNN-backed object detection model. Specifically, to reduce the parameter count, we first design an improved FasterNet module based on the Partial Convolution (PConv) operator. Then, to enhance convergence performance, we improve the loss function based on the efficient complete intersection over the union. Based on this, a flexible variable-length encoding is proposed, and the corresponding reproduction operators are designed. Related experimental results confirm that the proposed approach can achieve better fault detection results and improve by 2.6% in mean precision at 50 (mAP50) compared to the existing methods. Additionally, compared to training with the YOLOv8n model, the YOLOBFE model reduces the training parameters by 933,937 and decreases the GFLOPS (Giga Floating Point Operations Per Second) by 1.1.Keywords
Cite This Article
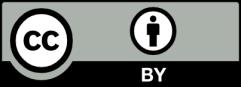