Open Access
ARTICLE
Generating Factual Text via Entailment Recognition Task
School of Cyber Science, Sichuan University, Chengdu, 610207, China
* Corresponding Author: Jiayong Liu. Email:
Computers, Materials & Continua 2024, 80(1), 547-565. https://doi.org/10.32604/cmc.2024.051745
Received 14 March 2024; Accepted 14 May 2024; Issue published 18 July 2024
Abstract
Generating diverse and factual text is challenging and is receiving increasing attention. By sampling from the latent space, variational autoencoder-based models have recently enhanced the diversity of generated text. However, existing research predominantly depends on summarization models to offer paragraph-level semantic information for enhancing factual correctness. The challenge lies in effectively generating factual text using sentence-level variational autoencoder-based models. In this paper, a novel model called fact-aware conditional variational autoencoder is proposed to balance the factual correctness and diversity of generated text. Specifically, our model encodes the input sentences and uses them as facts to build a conditional variational autoencoder network. By training a conditional variational autoencoder network, the model is enabled to generate text based on input facts. Building upon this foundation, the input text is passed to the discriminator along with the generated text. By employing adversarial training, the model is encouraged to generate text that is indistinguishable to the discriminator, thereby enhancing the quality of the generated text. To further improve the factual correctness, inspired by the natural language inference system, the entailment recognition task is introduced to be trained together with the discriminator via multi-task learning. Moreover, based on the entailment recognition results, a penalty term is further proposed to reconstruct the loss of our model, forcing the generator to generate text consistent with the facts. Experimental results demonstrate that compared with competitive models, our model has achieved substantial improvements in both the quality and factual correctness of the text, despite only sacrificing a small amount of diversity. Furthermore, when considering a comprehensive evaluation of diversity and quality metrics, our model has also demonstrated the best performance.Keywords
Cite This Article
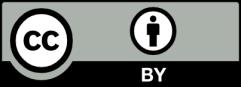
This work is licensed under a Creative Commons Attribution 4.0 International License , which permits unrestricted use, distribution, and reproduction in any medium, provided the original work is properly cited.