Open Access
ARTICLE
A New Speed Limit Recognition Methodology Based on Ensemble Learning: Hardware Validation
1 ESME, ESME Research Lab, Ivry Sur Seine, 94200, France
2 CEM, Lab ENIS, University of Sfax, Sfax, 3038, Tunisia
* Corresponding Authors: Mohamed Karray. Email: ; Nesrine Triki. Email:
Computers, Materials & Continua 2024, 80(1), 119-138. https://doi.org/10.32604/cmc.2024.051562
Received 08 March 2024; Accepted 01 June 2024; Issue published 18 July 2024
Abstract
Advanced Driver Assistance Systems (ADAS) technologies can assist drivers or be part of automatic driving systems to support the driving process and improve the level of safety and comfort on the road. Traffic Sign Recognition System (TSRS) is one of the most important components of ADAS. Among the challenges with TSRS is being able to recognize road signs with the highest accuracy and the shortest processing time. Accordingly, this paper introduces a new real time methodology recognizing Speed Limit Signs based on a trio of developed modules. Firstly, the Speed Limit Detection (SLD) module uses the Haar Cascade technique to generate a new SL detector in order to localize SL signs within captured frames. Secondly, the Speed Limit Classification (SLC) module, featuring machine learning classifiers alongside a newly developed model called DeepSL, harnesses the power of a CNN architecture to extract intricate features from speed limit sign images, ensuring efficient and precise recognition. In addition, a new Speed Limit Classifiers Fusion (SLCF) module has been developed by combining trained ML classifiers and the DeepSL model by using the Dempster-Shafer theory of belief functions and ensemble learning’s voting technique. Through rigorous software and hardware validation processes, the proposed methodology has achieved highly significant F1 scores of 99.98% and 99.96% for DS theory and the voting method, respectively. Furthermore, a prototype encompassing all components demonstrates outstanding reliability and efficacy, with processing times of 150 ms for the Raspberry Pi board and 81.5 ms for the Nano Jetson board, marking a significant advancement in TSRS technology.Keywords
Cite This Article
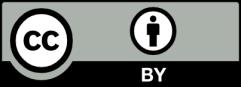
This work is licensed under a Creative Commons Attribution 4.0 International License , which permits unrestricted use, distribution, and reproduction in any medium, provided the original work is properly cited.