Open Access
ARTICLE
Cloud-Edge Collaborative Federated GAN Based Data Processing for IoT-Empowered Multi-Flow Integrated Energy Aggregation Dispatch
Electric Power Dispatching Control Center, Guangdong Power Grid Co., Ltd., Guangzhou, 510030, China
* Corresponding Author: Zhan Shi. Email:
Computers, Materials & Continua 2024, 80(1), 973-994. https://doi.org/10.32604/cmc.2024.051530
Received 07 March 2024; Accepted 27 May 2024; Issue published 18 July 2024
Abstract
The convergence of Internet of Things (IoT), 5G, and cloud collaboration offers tailored solutions to the rigorous demands of multi-flow integrated energy aggregation dispatch data processing. While generative adversarial networks (GANs) are instrumental in resource scheduling, their application in this domain is impeded by challenges such as convergence speed, inferior optimality searching capability, and the inability to learn from failed decision making feedbacks. Therefore, a cloud-edge collaborative federated GAN-based communication and computing resource scheduling algorithm with long-term constraint violation sensitiveness is proposed to address these challenges. The proposed algorithm facilitates real-time, energy-efficient data processing by optimizing transmission power control, data migration, and computing resource allocation. It employs federated learning for global parameter aggregation to enhance GAN parameter updating and dynamically adjusts GAN learning rates and global aggregation weights based on energy consumption constraint violations. Simulation results indicate that the proposed algorithm effectively reduces data processing latency, energy consumption, and convergence time.Keywords
Cite This Article
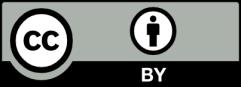
This work is licensed under a Creative Commons Attribution 4.0 International License , which permits unrestricted use, distribution, and reproduction in any medium, provided the original work is properly cited.