Open Access
ARTICLE
Enhancing Tea Leaf Disease Identification with Lightweight MobileNetV2
1 College of Big Data and Information Engineering, Guizhou University, Guiyang, 550025, China
2 Power Semiconductor Device Reliability Engineering Center of the Ministry of Education, Guiyang, 550025, China
3 State Key Laboratory of Public Big Data, Guizhou University, Guiyang, 550025, China
4 The Mushroom Research Centre, Guizhou University, Guiyang, 550025, China
5 The Engineering Research Center of Southwest Bio-Pharmaceutical Resources, Ministry of Education, Guizhou University, Guiyang, 550025, China
* Corresponding Author: Benliang Xie. Email:
Computers, Materials & Continua 2024, 80(1), 679-694. https://doi.org/10.32604/cmc.2024.051526
Received 07 March 2024; Accepted 16 May 2024; Issue published 18 July 2024
Abstract
Diseases in tea trees can result in significant losses in both the quality and quantity of tea production. Regular monitoring can help to prevent the occurrence of large-scale diseases in tea plantations. However, existing methods face challenges such as a high number of parameters and low recognition accuracy, which hinders their application in tea plantation monitoring equipment. This paper presents a lightweight I-MobileNetV2 model for identifying diseases in tea leaves, to address these challenges. The proposed method first embeds a Coordinate Attention (CA) module into the original MobileNetV2 network, enabling the model to locate disease regions accurately. Secondly, a Multi-branch Parallel Convolution (MPC) module is employed to extract disease features across multiple scales, improving the model’s adaptability to different disease scales. Finally, the AutoML for Model Compression (AMC) is used to compress the model and reduce computational complexity. Experimental results indicate that our proposed algorithm attains an average accuracy of 96.12% on our self-built tea leaf disease dataset, surpassing the original MobileNetV2 by 1.91%. Furthermore, the number of model parameters have been reduced by 40%, making it more suitable for practical application in tea plantation environments.Keywords
Cite This Article
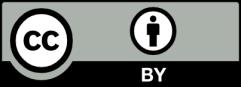
This work is licensed under a Creative Commons Attribution 4.0 International License , which permits unrestricted use, distribution, and reproduction in any medium, provided the original work is properly cited.