Open Access
ARTICLE
Target Detection on Water Surfaces Using Fusion of Camera and LiDAR Based Information
College of Engineering Science and Technology, Shanghai Ocean University, Shanghai, 201306, China
* Corresponding Author: Jia Xie. Email:
(This article belongs to the Special Issue: Multimodal Learning in Image Processing)
Computers, Materials & Continua 2024, 80(1), 467-486. https://doi.org/10.32604/cmc.2024.051426
Received 05 March 2024; Accepted 24 April 2024; Issue published 18 July 2024
Abstract
To address the challenges of missed detections in water surface target detection using solely visual algorithms in unmanned surface vehicle (USV) perception, this paper proposes a method based on the fusion of visual and LiDAR point-cloud projection for water surface target detection. Firstly, the visual recognition component employs an improved YOLOv7 algorithm based on a self-built dataset for the detection of water surface targets. This algorithm modifies the original YOLOv7 architecture to a Slim-Neck structure, addressing the problem of excessive redundant information during feature extraction in the original YOLOv7 network model. Simultaneously, this modification simplifies the computational burden of the detector, reduces inference time, and maintains accuracy. Secondly, to tackle the issue of sample imbalance in the self-built dataset, slide loss function is introduced. Finally, this paper replaces the original Complete Intersection over Union (CIoU) loss function with the Minimum Point Distance Intersection over Union (MPDIoU) loss function in the YOLOv7 algorithm, which accelerates model learning and enhances robustness. To mitigate the problem of missed recognitions caused by complex water surface conditions in purely visual algorithms, this paper further adopts the fusion of LiDAR and camera data, projecting the three-dimensional point-cloud data from LiDAR onto a two-dimensional pixel plane. This significantly reduces the rate of missed detections for water surface targets.Keywords
Cite This Article
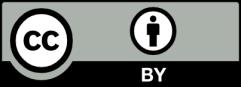
This work is licensed under a Creative Commons Attribution 4.0 International License , which permits unrestricted use, distribution, and reproduction in any medium, provided the original work is properly cited.