Open Access
ARTICLE
Optimized Binary Neural Networks for Road Anomaly Detection: A TinyML Approach on Edge Devices
1 School of Information Engineering, Chang’an University, Xi’an, 710064, China
2 School of Information Engineering, Xi’an Eurasia University, Xi’an, 710065, China
3 School of Electrical and Electronic Engineering, Wenzhou University, Wenzhou, 325035, China
* Corresponding Authors: Weixing Wang. Email: ; Limin Li. Email:
(This article belongs to the Special Issue: Data and Image Processing in Intelligent Information Systems)
Computers, Materials & Continua 2024, 80(1), 527-546. https://doi.org/10.32604/cmc.2024.051147
Received 28 February 2024; Accepted 14 May 2024; Issue published 18 July 2024
Abstract
Integrating Tiny Machine Learning (TinyML) with edge computing in remotely sensed images enhances the capabilities of road anomaly detection on a broader level. Constrained devices efficiently implement a Binary Neural Network (BNN) for road feature extraction, utilizing quantization and compression through a pruning strategy. The modifications resulted in a 28-fold decrease in memory usage and a 25% enhancement in inference speed while only experiencing a 2.5% decrease in accuracy. It showcases its superiority over conventional detection algorithms in different road image scenarios. Although constrained by computer resources and training datasets, our results indicate opportunities for future research, demonstrating that quantization and focused optimization can significantly improve machine learning models’ accuracy and operational efficiency. ARM Cortex-M0 gives practical feasibility and substantial benefits while deploying our optimized BNN model on this low-power device: Advanced machine learning in edge computing. The analysis work delves into the educational significance of TinyML and its essential function in analyzing road networks using remote sensing, suggesting ways to improve smart city frameworks in road network assessment, traffic management, and autonomous vehicle navigation systems by emphasizing the importance of new technologies for maintaining and safeguarding road networks.Keywords
Cite This Article
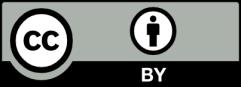