Open Access
ARTICLE
Learning Dual-Layer User Representation for Enhanced Item Recommendation
1 Applied Research Center of Artificial Intelligence, Wuhan College, Wuhan, 430212, China
2 College of Computer Science, South-Central MINZU University, Wuhan, 430074, China
3 Unmanned. Company, Riyadh, 11564, Saudi Arabia
* Corresponding Author: Jin Xie. Email:
(This article belongs to the Special Issue: The Next-generation Deep Learning Approaches to Emerging Real-world Applications)
Computers, Materials & Continua 2024, 80(1), 949-971. https://doi.org/10.32604/cmc.2024.051046
Received 26 February 2024; Accepted 23 May 2024; Issue published 18 July 2024
Abstract
User representation learning is crucial for capturing different user preferences, but it is also critical challenging because user intentions are latent and dispersed in complex and different patterns of user-generated data, and thus cannot be measured directly. Text-based data models can learn user representations by mining latent semantics, which is beneficial to enhancing the semantic function of user representations. However, these technologies only extract common features in historical records and cannot represent changes in user intentions. However, sequential feature can express the user’s interests and intentions that change time by time. But the sequential recommendation results based on the user representation of the item lack the interpretability of preference factors. To address these issues, we propose in this paper a novel model with Dual-Layer User Representation, named DLUR, where the user’s intention is learned based on two different layer representations. Specifically, the latent semantic layer adds an interactive layer based on Transformer to extract keywords and key sentences in the text and serve as a basis for interpretation. The sequence layer uses the Transformer model to encode the user’s preference intention to clarify changes in the user’s intention. Therefore, this dual-layer user mode is more comprehensive than a single text mode or sequence mode and can effectually improve the performance of recommendations. Our extensive experiments on five benchmark datasets demonstrate DLUR’s performance over state-of-the-art recommendation models. In addition, DLUR’s ability to explain recommendation results is also demonstrated through some specific cases.Keywords
Cite This Article
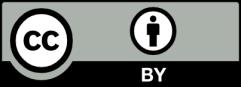