Open Access
ARTICLE
Contemporary Study for Detection of COVID-19 Using Machine Learning with Explainable AI
1 Department of Computer Science, University of Karachi, Karachi, 75270, Pakistan
2 Department of Computing, Faculty of Engineering Science and Technology, Hamdard University, Karachi, 75540, Pakistan
3 Department of Health Informatics, College of Applied Medical Sciences, Qassim University, Qassim, 51452, Saudi Arabia
4 Department of Computer Science, College of Computer Science, King Khalid University, Abha, 61421, Saudi Arabia
* Corresponding Author: Sulaiman Sulmi Almutairi. Email:
(This article belongs to the Special Issue: Medical Imaging Based Disease Diagnosis Using AI)
Computers, Materials & Continua 2024, 80(1), 1075-1104. https://doi.org/10.32604/cmc.2024.050913
Received 22 February 2024; Accepted 23 May 2024; Issue published 18 July 2024
Abstract
The prompt spread of COVID-19 has emphasized the necessity for effective and precise diagnostic tools. In this article, a hybrid approach in terms of datasets as well as the methodology by utilizing a previously unexplored dataset obtained from a private hospital for detecting COVID-19, pneumonia, and normal conditions in chest X-ray images (CXIs) is proposed coupled with Explainable Artificial Intelligence (XAI). Our study leverages less preprocessing with pre-trained cutting-edge models like InceptionV3, VGG16, and VGG19 that excel in the task of feature extraction. The methodology is further enhanced by the inclusion of the t-SNE (t-Distributed Stochastic Neighbor Embedding) technique for visualizing the extracted image features and Contrast Limited Adaptive Histogram Equalization (CLAHE) to improve images before extraction of features. Additionally, an Attention Mechanism is utilized, which helps clarify how the model makes decisions, which builds trust in artificial intelligence (AI) systems. To evaluate the effectiveness of the proposed approach, both benchmark datasets and a private dataset obtained with permissions from Jinnah Postgraduate Medical Center (JPMC) in Karachi, Pakistan, are utilized. In 12 experiments, VGG19 showcased remarkable performance in the hybrid dataset approach, achieving 100% accuracy in COVID-19 vs. pneumonia classification and 97% in distinguishing normal cases. Overall, across all classes, the approach achieved 98% accuracy, demonstrating its efficiency in detecting COVID-19 and differentiating it from other chest disorders (Pneumonia and healthy) while also providing insights into the decision-making process of the models.Keywords
Cite This Article
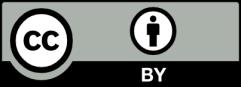
This work is licensed under a Creative Commons Attribution 4.0 International License , which permits unrestricted use, distribution, and reproduction in any medium, provided the original work is properly cited.