Open Access
REVIEW
An Integrated Analysis of Yield Prediction Models: A Comprehensive Review of Advancements and Challenges
1 School of Computer Science and Engineering, Galgotias University, Greater Noida, 203201, India
2 Department of Applied Data Science, Noroff University College, Kristiansand, 4612, Norway
3 Artificial Intelligence Research Center (AIRC), Ajman University, Ajman, 346, United Arab Emirates
4 MEU Research Unit, Middle East University, Amman, 11831, Jordan
5 Department of Computer Science, College of Computing, Khon Kaen University, Khon Kaen, 40002, Thailand
* Corresponding Author: Seifedine Kadry. Email:
Computers, Materials & Continua 2024, 80(1), 389-425. https://doi.org/10.32604/cmc.2024.050240
Received 13 January 2024; Accepted 11 May 2024; Issue published 18 July 2024
Abstract
The growing global requirement for food and the need for sustainable farming in an era of a changing climate and scarce resources have inspired substantial crop yield prediction research. Deep learning (DL) and machine learning (ML) models effectively deal with such challenges. This research paper comprehensively analyses recent advancements in crop yield prediction from January 2016 to March 2024. In addition, it analyses the effectiveness of various input parameters considered in crop yield prediction models. We conducted an in-depth search and gathered studies that employed crop modeling and AI-based methods to predict crop yield. The total number of articles reviewed for crop yield prediction using ML, meta-modeling (Crop models coupled with ML/DL), and DL-based prediction models and input parameter selection is 125. We conduct the research by setting up five objectives for this research and discussing them after analyzing the selected research papers. Each study is assessed based on the crop type, input parameters employed for prediction, the modeling techniques adopted, and the evaluation metrics used for estimating model performance. We also discuss the ethical and social impacts of AI on agriculture. However, various approaches presented in the scientific literature have delivered impressive predictions, they are complicated due to intricate, multifactorial influences on crop growth and the need for accurate data-driven models. Therefore, thorough research is required to deal with challenges in predicting agricultural output.Keywords
Cite This Article
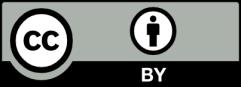
This work is licensed under a Creative Commons Attribution 4.0 International License , which permits unrestricted use, distribution, and reproduction in any medium, provided the original work is properly cited.