Open Access
ARTICLE
Automatic Rule Discovery for Data Transformation Using Fusion of Diversified Feature Formats
1 Department of CSE, Jawaharlal Nehru Technological University, Anantapur, 515002, India
2 Department of CSE, Marri Laxman Reddy Institute of Technology and Management, Hyderabad, 500043, India
3 Department of Information Technology, Mahatma Gandhi Institute of Technology, Hyderabad, 500075, India
* Corresponding Author: G. Sunil Santhosh Kumar. Email:
Computers, Materials & Continua 2024, 80(1), 695-713. https://doi.org/10.32604/cmc.2024.050143
Received 29 January 2024; Accepted 14 May 2024; Issue published 18 July 2024
Abstract
This article presents an innovative approach to automatic rule discovery for data transformation tasks leveraging XGBoost, a machine learning algorithm renowned for its efficiency and performance. The framework proposed herein utilizes the fusion of diversified feature formats, specifically, metadata, textual, and pattern features. The goal is to enhance the system’s ability to discern and generalize transformation rules from source to destination formats in varied contexts. Firstly, the article delves into the methodology for extracting these distinct features from raw data and the pre-processing steps undertaken to prepare the data for the model. Subsequent sections expound on the mechanism of feature optimization using Recursive Feature Elimination (RFE) with linear regression, aiming to retain the most contributive features and eliminate redundant or less significant ones. The core of the research revolves around the deployment of the XGBoost model for training, using the prepared and optimized feature sets. The article presents a detailed overview of the mathematical model and algorithmic steps behind this procedure. Finally, the process of rule discovery (prediction phase) by the trained XGBoost model is explained, underscoring its role in real-time, automated data transformations. By employing machine learning and particularly, the XGBoost model in the context of Business Rule Engine (BRE) data transformation, the article underscores a paradigm shift towards more scalable, efficient, and less human-dependent data transformation systems. This research opens doors for further exploration into automated rule discovery systems and their applications in various sectors.Keywords
Cite This Article
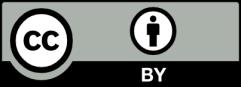