Open Access
ARTICLE
5G Resource Allocation Using Feature Selection and Greylag Goose Optimization Algorithm
1 Department of Computer Sciences, College of Computer and Information Sciences, Princess Nourah bint Abdulrahman University, P.O. Box 84428, Riyadh, 11671, Saudi Arabia
2 Computer Science and Intelligent Systems Research Center, Blacksburg, VA 24060, USA
* Corresponding Author: S. K. Towfek. Email:
Computers, Materials & Continua 2024, 80(1), 1179-1201. https://doi.org/10.32604/cmc.2024.049874
Received 21 January 2024; Accepted 29 May 2024; Issue published 18 July 2024
Abstract
In the contemporary world of highly efficient technological development, fifth-generation technology (5G) is seen as a vital step forward with theoretical maximum download speeds of up to twenty gigabits per second (Gbps). As far as the current implementations are concerned, they are at the level of slightly below 1 Gbps, but this allowed a great leap forward from fourth generation technology (4G), as well as enabling significantly reduced latency, making 5G an absolute necessity for applications such as gaming, virtual conferencing, and other interactive electronic processes. Prospects of this change are not limited to connectivity alone; it urges operators to refine their business strategies and offers users better and improved digital solutions. An essential factor is optimization and the application of artificial intelligence throughout the general arrangement of intricate and detailed 5G lines. Integrating Binary Greylag Goose Optimization (bGGO) to achieve a significant reduction in the feature set while maintaining or improving model performance, leading to more efficient and effective 5G network management, and Greylag Goose Optimization (GGO) increases the efficiency of the machine learning models. Thus, the model performs and yields more accurate results. This work proposes a new method to schedule the resources in the next generation, 5G, based on a feature selection using GGO and a regression model that is an ensemble of K-Nearest Neighbors (KNN), Gradient Boosting, and Extra Trees algorithms. The ensemble model shows better prediction performance with the coefficient of determination R squared value equal to. 99348. The proposed framework is supported by several Statistical analyses, such as the Wilcoxon signed-rank test. Some of the benefits of this study are the introduction of new efficient optimization algorithms, the selection of features and more reliable ensemble models which improve the efficiency of 5G technology.Keywords
Cite This Article
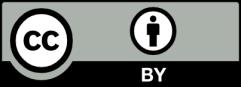