Open Access
ARTICLE
UNet Based on Multi-Object Segmentation and Convolution Neural Network for Object Recognition
1 Department of Information Systems, College of Computer and Information Sciences, Princess Nourah bint Abdulrahman University, P.O. Box 84428, Riyadh, 11671, Saudi Arabia
2 Department of Computer Science, Air University, Islamabad, 44000, Pakistan
3 Department of Computer Science, College of Computer Science and Information System, Najran University, Najran, 55461, Saudi Arabia
4 Department of Computer Sciences, Faculty of Computing and Information Technology, Northern Border University, Rafha, 91911, Saudi Arabia
5 Information Technology Department, College of Computing and Informatics, Saudi Electronic University, Riyadh, 13316, Saudi Arabia
6 Department of Computer Engineering, Korea Polytechnic University, Siheung-si, Gyeonggi-do, 237, Republic of Korea
* Corresponding Author: Jeongmin Park. Email:
(This article belongs to the Special Issue: Multimodal Learning in Image Processing)
Computers, Materials & Continua 2024, 80(1), 1563-1580. https://doi.org/10.32604/cmc.2024.049333
Received 03 January 2024; Accepted 25 April 2024; Issue published 18 July 2024
Abstract
The recent advancements in vision technology have had a significant impact on our ability to identify multiple objects and understand complex scenes. Various technologies, such as augmented reality-driven scene integration, robotic navigation, autonomous driving, and guided tour systems, heavily rely on this type of scene comprehension. This paper presents a novel segmentation approach based on the UNet network model, aimed at recognizing multiple objects within an image. The methodology begins with the acquisition and preprocessing of the image, followed by segmentation using the fine-tuned UNet architecture. Afterward, we use an annotation tool to accurately label the segmented regions. Upon labeling, significant features are extracted from these segmented objects, encompassing KAZE (Accelerated Segmentation and Extraction) features, energy-based edge detection, frequency-based, and blob characteristics. For the classification stage, a convolution neural network (CNN) is employed. This comprehensive methodology demonstrates a robust framework for achieving accurate and efficient recognition of multiple objects in images. The experimental results, which include complex object datasets like MSRC-v2 and PASCAL-VOC12, have been documented. After analyzing the experimental results, it was found that the PASCAL-VOC12 dataset achieved an accuracy rate of 95%, while the MSRC-v2 dataset achieved an accuracy of 89%. The evaluation performed on these diverse datasets highlights a notably impressive level of performance.Keywords
Cite This Article
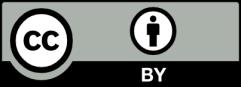
This work is licensed under a Creative Commons Attribution 4.0 International License , which permits unrestricted use, distribution, and reproduction in any medium, provided the original work is properly cited.