Open Access
ARTICLE
Urban Electric Vehicle Charging Station Placement Optimization with Graylag Goose Optimization Voting Classifier
1 Department of Computer Sciences, College of Computer and Information Sciences, Princess Nourah bint Abdulrahman University, P.O. Box 84428, Riyadh, 11671, Saudi Arabia
2 Department of Communications and Electronics, Delta Higher Institute for Engineering and Technology, Mansoura, 35511, Egypt
3 Faculty of Artificial Intelligence, Delta University for Science and Technology, Mansoura, 35712, Egypt
4 Computer Engineering and Control Systems Department, Faculty of Engineering, Mansoura University, Mansoura, 35516, Egypt
* Corresponding Author: El-Sayed M. El-kenawy. Email:
Computers, Materials & Continua 2024, 80(1), 1163-1177. https://doi.org/10.32604/cmc.2024.049001
Received 24 December 2023; Accepted 03 June 2024; Issue published 18 July 2024
Abstract
To reduce the negative effects that conventional modes of transportation have on the environment, researchers are working to increase the use of electric vehicles. The demand for environmentally friendly transportation may be hampered by obstacles such as a restricted range and extended rates of recharge. The establishment of urban charging infrastructure that includes both fast and ultra-fast terminals is essential to address this issue. Nevertheless, the powering of these terminals presents challenges because of the high energy requirements, which may influence the quality of service. Modelling the maximum hourly capacity of each station based on its geographic location is necessary to arrive at an accurate estimation of the resources required for charging infrastructure. It is vital to do an analysis of specific regional traffic patterns, such as road networks, route details, junction density, and economic zones, rather than making arbitrary conclusions about traffic patterns. When vehicle traffic is simulated using this data and other variables, it is possible to detect limits in the design of the current traffic engineering system. Initially, the binary graylag goose optimization (bGGO) algorithm is utilized for the purpose of feature selection. Subsequently, the graylag goose optimization (GGO) algorithm is utilized as a voting classifier as a decision algorithm to allocate demand to charging stations while taking into consideration the cost variable of traffic congestion. Based on the results of the analysis of variance (ANOVA), a comprehensive summary of the components that contribute to the observed variability in the dataset is provided. The results of the Wilcoxon Signed Rank Test compare the actual median accuracy values of several different algorithms, such as the voting GGO algorithm, the voting grey wolf optimization algorithm (GWO), the voting whale optimization algorithm (WOA), the voting particle swarm optimization (PSO), the voting firefly algorithm (FA), and the voting genetic algorithm (GA), to the theoretical median that would be expected that there is no difference.Keywords
Cite This Article
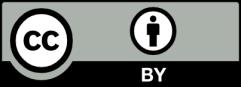