Open Access
ARTICLE
Refined Anam-Net: Lightweight Deep Learning Model for Improved Segmentation Performance of Optic Cup and Disc for Glaucoma Diagnosis
Department of Computer Engineering, College of Computer and Information Sciences, King Saud University, P.O. Box 51178, Riyadh, 11543, Saudi Arabia
* Corresponding Author: Khursheed Aurangzeb. Email:
Computers, Materials & Continua 2024, 80(1), 1381-1405. https://doi.org/10.32604/cmc.2024.048987
Received 23 December 2023; Accepted 22 May 2024; Issue published 18 July 2024
Abstract
In this work, we aim to introduce some modifications to the Anam-Net deep neural network (DNN) model for segmenting optic cup (OC) and optic disc (OD) in retinal fundus images to estimate the cup-to-disc ratio (CDR). The CDR is a reliable measure for the early diagnosis of Glaucoma. In this study, we developed a lightweight DNN model for OC and OD segmentation in retinal fundus images. Our DNN model is based on modifications to Anam-Net, incorporating an anamorphic depth embedding block. To reduce computational complexity, we employ a fixed filter size for all convolution layers in the encoder and decoder stages as the network deepens. This modification significantly reduces the number of trainable parameters, making the model lightweight and suitable for resource-constrained applications. We evaluate the performance of the developed model using two publicly available retinal image databases, namely RIM-ONE and Drishti-GS. The results demonstrate promising OC segmentation performance across most standard evaluation metrics while achieving analogous results for OD segmentation. We used two retinal fundus image databases named RIM-ONE and Drishti-GS that contained 159 images and 101 retinal images, respectively. For OD segmentation using the RIM-ONE we obtain an f1-score (F1), Jaccard coefficient (JC), and overlapping error (OE) of 0.950, 0.9219, and 0.0781, respectively. Similarly, for OC segmentation using the same databases, we achieve scores of 0.8481 (F1), 0.7428 (JC), and 0.2572 (OE). Based on these experimental results and the significantly lower number of trainable parameters, we conclude that the developed model is highly suitable for the early diagnosis of glaucoma by accurately estimating the CDR.Keywords
Cite This Article
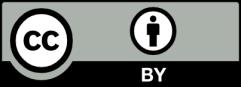