Open Access
ARTICLE
A Machine Learning Approach to Cyberbullying Detection in Arabic Tweets
1 Department of Computer Science, College of Computer Science and Information Technology, Imam Abdulrahman Bin Faisal University, P.O. Box 1982, Dammam, 31441, Saudi Arabia
2 Department of Computer Information Systems, College of Computer Science and Information Technology, Imam Abdulrahman Bin Faisal University, P.O. Box 1982, Dammam, 31441, Saudi Arabia
3 Department of Networks and Communications, College of Computer Science and Information Technology, Imam Abdulrahman Bin Faisal University, P.O. Box 1982, Dammam, 31441, Saudi Arabia
* Corresponding Author: Atta Rahman. Email:
Computers, Materials & Continua 2024, 80(1), 1033-1054. https://doi.org/10.32604/cmc.2024.048003
Received 24 November 2023; Accepted 28 May 2024; Issue published 18 July 2024
Abstract
With the rapid growth of internet usage, a new situation has been created that enables practicing bullying. Cyberbullying has increased over the past decade, and it has the same adverse effects as face-to-face bullying, like anger, sadness, anxiety, and fear. With the anonymity people get on the internet, they tend to be more aggressive and express their emotions freely without considering the effects, which can be a reason for the increase in cyberbullying and it is the main motive behind the current study. This study presents a thorough background of cyberbullying and the techniques used to collect, preprocess, and analyze the datasets. Moreover, a comprehensive review of the literature has been conducted to figure out research gaps and effective techniques and practices in cyberbullying detection in various languages, and it was deduced that there is significant room for improvement in the Arabic language. As a result, the current study focuses on the investigation of shortlisted machine learning algorithms in natural language processing (NLP) for the classification of Arabic datasets duly collected from Twitter (also known as X). In this regard, support vector machine (SVM), Naïve Bayes (NB), Random Forest (RF), Logistic regression (LR), Bootstrap aggregating (Bagging), Gradient Boosting (GBoost), Light Gradient Boosting Machine (LightGBM), Adaptive Boosting (AdaBoost), and eXtreme Gradient Boosting (XGBoost) were shortlisted and investigated due to their effectiveness in the similar problems. Finally, the scheme was evaluated by well-known performance measures like accuracy, precision, Recall, and F1-score. Consequently, XGBoost exhibited the best performance with 89.95% accuracy, which is promising compared to the state-of-the-art.Keywords
Cite This Article
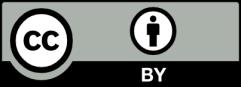
This work is licensed under a Creative Commons Attribution 4.0 International License , which permits unrestricted use, distribution, and reproduction in any medium, provided the original work is properly cited.