Open Access
ARTICLE
Research on Multi-Scale Feature Fusion Network Algorithm Based on Brain Tumor Medical Image Classification
1 Graduate School, China Academy of Engineering Physics, Beijing, 100000, China
2 Department of Computational Mathematics, Beijing Institute of Applied Physics and Computational Mathematics, Beijing, 100000, China
3 National Key Laboratory of Computational Physics, Beijing, 100088, China
4 Shanghai Zhangjiang Institute of Mathematics, Shanghai, 201203, China
* Corresponding Author: Junping Yin. Email:
(This article belongs to the Special Issue: Deep Learning in Computer-Aided Diagnosis Based on Medical Image)
Computers, Materials & Continua 2024, 79(3), 5313-5333. https://doi.org/10.32604/cmc.2024.052060
Received 21 March 2024; Accepted 11 May 2024; Issue published 20 June 2024
Abstract
Gliomas have the highest mortality rate of all brain tumors. Correctly classifying the glioma risk period can help doctors make reasonable treatment plans and improve patients’ survival rates. This paper proposes a hierarchical multi-scale attention feature fusion medical image classification network (HMAC-Net), which effectively combines global features and local features. The network framework consists of three parallel layers: The global feature extraction layer, the local feature extraction layer, and the multi-scale feature fusion layer. A linear sparse attention mechanism is designed in the global feature extraction layer to reduce information redundancy. In the local feature extraction layer, a bilateral local attention mechanism is introduced to improve the extraction of relevant information between adjacent slices. In the multi-scale feature fusion layer, a channel fusion block combining convolutional attention mechanism and residual inverse multi-layer perceptron is proposed to prevent gradient disappearance and network degradation and improve feature representation capability. The double-branch iterative multi-scale classification block is used to improve the classification performance. On the brain glioma risk grading dataset, the results of the ablation experiment and comparison experiment show that the proposed HMAC-Net has the best performance in both qualitative analysis of heat maps and quantitative analysis of evaluation indicators. On the dataset of skin cancer classification, the generalization experiment results show that the proposed HMAC-Net has a good generalization effect.Keywords
Cite This Article
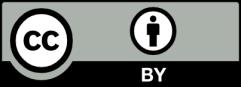
This work is licensed under a Creative Commons Attribution 4.0 International License , which permits unrestricted use, distribution, and reproduction in any medium, provided the original work is properly cited.