Open Access
ARTICLE
Optimised CNN Architectures for Handwritten Arabic Character Recognition
Department of Computer Science, The World Islamic Sciences and Education University, Amman, 1101-11947, Jordan
* Corresponding Author: Salah Alghyaline. Email:
Computers, Materials & Continua 2024, 79(3), 4905-4924. https://doi.org/10.32604/cmc.2024.052016
Received 20 March 2024; Accepted 07 May 2024; Issue published 20 June 2024
Abstract
Handwritten character recognition is considered challenging compared with machine-printed characters due to the different human writing styles. Arabic is morphologically rich, and its characters have a high similarity. The Arabic language includes 28 characters. Each character has up to four shapes according to its location in the word (at the beginning, middle, end, and isolated). This paper proposed 12 CNN architectures for recognizing handwritten Arabic characters. The proposed architectures were derived from the popular CNN architectures, such as VGG, ResNet, and Inception, to make them applicable to recognizing character-size images. The experimental results on three well-known datasets showed that the proposed architectures significantly enhanced the recognition rate compared to the baseline models. The experiments showed that data augmentation improved the models’ accuracies on all tested datasets. The proposed model outperformed most of the existing approaches. The best achieved results were 93.05%, 98.30%, and 96.88% on the HIJJA, AHCD, and AIA9K datasets.Keywords
Cite This Article
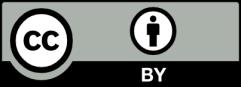
This work is licensed under a Creative Commons Attribution 4.0 International License , which permits unrestricted use, distribution, and reproduction in any medium, provided the original work is properly cited.