Open Access
ARTICLE
The Lightweight Edge-Side Fault Diagnosis Approach Based on Spiking Neural Network
State Key Laboratory of Networking and Switching Technology, Beijing University of Posts and Telecommunications, Beijing, 100876, China
* Corresponding Author: Yang Yang. Email:
Computers, Materials & Continua 2024, 79(3), 4883-4904. https://doi.org/10.32604/cmc.2024.051860
Received 17 March 2024; Accepted 28 April 2024; Issue published 20 June 2024
Abstract
Network fault diagnosis methods play a vital role in maintaining network service quality and enhancing user experience as an integral component of intelligent network management. Considering the unique characteristics of edge networks, such as limited resources, complex network faults, and the need for high real-time performance, enhancing and optimizing existing network fault diagnosis methods is necessary. Therefore, this paper proposes the lightweight edge-side fault diagnosis approach based on a spiking neural network (LSNN). Firstly, we use the Izhikevich neurons model to replace the Leaky Integrate and Fire (LIF) neurons model in the LSNN model. Izhikevich neurons inherit the simplicity of LIF neurons but also possess richer behavioral characteristics and flexibility to handle diverse data inputs. Inspired by Fast Spiking Interneurons (FSIs) with a high-frequency firing pattern, we use the parameters of FSIs. Secondly, inspired by the connection mode based on spiking dynamics in the basal ganglia (BG) area of the brain, we propose the pruning approach based on the FSIs of the BG in LSNN to improve computational efficiency and reduce the demand for computing resources and energy consumption. Furthermore, we propose a multiple iterative Dynamic Spike Timing Dependent Plasticity (DSTDP) algorithm to enhance the accuracy of the LSNN model. Experiments on two server fault datasets demonstrate significant precision, recall, and F1 improvements across three diagnosis dimensions. Simultaneously, lightweight indicators such as Params and FLOPs significantly reduced, showcasing the LSNN’s advanced performance and model efficiency. To conclude, experiment results on a pair of datasets indicate that the LSNN model surpasses traditional models and achieves cutting-edge outcomes in network fault diagnosis tasks.Keywords
Cite This Article
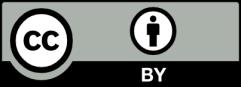
This work is licensed under a Creative Commons Attribution 4.0 International License , which permits unrestricted use, distribution, and reproduction in any medium, provided the original work is properly cited.