Open Access
ARTICLE
Joint Rain Streaks & Haze Removal Network for Object Detection
1 Department of Electronics and Communication Engineering, National Institute of Technology, Warangal, 506004, India
2 Department of Computer Science and Engineering, National Institute of Technology, Warangal, 506004, India
3 Department of Electronics and Communication Engineering, Indian Institute of Information Technology, Design and Manufacturing, Kurnool, 518008, India
* Corresponding Author: Prakash Kodali. Email:
Computers, Materials & Continua 2024, 79(3), 4683-4702. https://doi.org/10.32604/cmc.2024.051844
Received 16 March 2024; Accepted 25 April 2024; Issue published 20 June 2024
Abstract
In the realm of low-level vision tasks, such as image deraining and dehazing, restoring images distorted by adverse weather conditions remains a significant challenge. The emergence of abundant computational resources has driven the dominance of deep Convolutional Neural Networks (CNNs), supplanting traditional methods reliant on prior knowledge. However, the evolution of CNN architectures has tended towards increasing complexity, utilizing intricate structures to enhance performance, often at the expense of computational efficiency. In response, we propose the Selective Kernel Dense Residual M-shaped Network (SKDRMNet), a flexible solution adept at balancing computational efficiency with network accuracy. A key innovation is the incorporation of an M-shaped hierarchical structure, derived from the U-Net framework as M-Network (M-Net), within which the Selective Kernel Dense Residual Module (SDRM) is introduced to reinforce multi-scale semantic feature maps. Our methodology employs two sampling techniques-bilinear and pixel unshuffled and utilizes a multi-scale feature fusion approach to distil more robust spatial feature map information. During the reconstruction phase, feature maps of varying resolutions are seamlessly integrated, and the extracted features are effectively merged using the Selective Kernel Fusion Module (SKFM). Empirical results demonstrate the comprehensive superiority of SKDRMNet across both synthetic and real rain and haze datasets.Keywords
Cite This Article
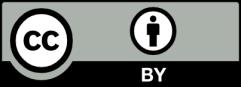