Open Access
ARTICLE
BDPartNet: Feature Decoupling and Reconstruction Fusion Network for Infrared and Visible Image
1 Department of Computer Science and Engineering, Chongqing University of Technology, Chongqing, 400054, China
2 Liangjiang Institute of Artificial Intelligence, Chongqing University of Technology, Chongqing, 400054, China
* Corresponding Author: Jianxun Zhang. Email:
(This article belongs to the Special Issue: Multimodal Learning in Image Processing)
Computers, Materials & Continua 2024, 79(3), 4621-4639. https://doi.org/10.32604/cmc.2024.051556
Received 08 March 2024; Accepted 17 April 2024; Issue published 20 June 2024
Abstract
While single-modal visible light images or infrared images provide limited information, infrared light captures significant thermal radiation data, whereas visible light excels in presenting detailed texture information. Combining images obtained from both modalities allows for leveraging their respective strengths and mitigating individual limitations, resulting in high-quality images with enhanced contrast and rich texture details. Such capabilities hold promising applications in advanced visual tasks including target detection, instance segmentation, military surveillance, pedestrian detection, among others. This paper introduces a novel approach, a dual-branch decomposition fusion network based on AutoEncoder (AE), which decomposes multi-modal features into intensity and texture information for enhanced fusion. Local contrast enhancement module (CEM) and texture detail enhancement module (DEM) are devised to process the decomposed images, followed by image fusion through the decoder. The proposed loss function ensures effective retention of key information from the source images of both modalities. Extensive comparisons and generalization experiments demonstrate the superior performance of our network in preserving pixel intensity distribution and retaining texture details. From the qualitative results, we can see the advantages of fusion details and local contrast. In the quantitative experiments, entropy (EN), mutual information (MI), structural similarity (SSIM) and other results have improved and exceeded the SOTA (State of the Art) model as a whole.Keywords
Cite This Article
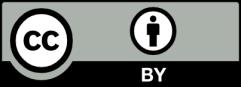