Open Access
ARTICLE
A Robust Approach for Multi Classification-Based Intrusion Detection through Stacking Deep Learning Models
Department of Information Technology, College of Computer and Information Sciences, Princess Nourah bint Abdulrahman University, Riyadh, 11671, Saudi Arabia
* Corresponding Author: Samia Allaoua Chelloug. Email:
Computers, Materials & Continua 2024, 79(3), 4845-4861. https://doi.org/10.32604/cmc.2024.051539
Received 08 March 2024; Accepted 29 April 2024; Issue published 20 June 2024
Abstract
Intrusion detection is a predominant task that monitors and protects the network infrastructure. Therefore, many datasets have been published and investigated by researchers to analyze and understand the problem of intrusion prediction and detection. In particular, the Network Security Laboratory-Knowledge Discovery in Databases (NSL-KDD) is an extensively used benchmark dataset for evaluating intrusion detection systems (IDSs) as it incorporates various network traffic attacks. It is worth mentioning that a large number of studies have tackled the problem of intrusion detection using machine learning models, but the performance of these models often decreases when evaluated on new attacks. This has led to the utilization of deep learning techniques, which have showcased significant potential for processing large datasets and therefore improving detection accuracy. For that reason, this paper focuses on the role of stacking deep learning models, including convolution neural network (CNN) and deep neural network (DNN) for improving the intrusion detection rate of the NSL-KDD dataset. Each base model is trained on the NSL-KDD dataset to extract significant features. Once the base models have been trained, the stacking process proceeds to the second stage, where a simple meta-model has been trained on the predictions generated from the proposed base models. The combination of the predictions allows the meta-model to distinguish different classes of attacks and increase the detection rate. Our experimental evaluations using the NSL-KDD dataset have shown the efficacy of stacking deep learning models for intrusion detection. The performance of the ensemble of base models, combined with the meta-model, exceeds the performance of individual models. Our stacking model has attained an accuracy of 99% and an average F1-score of 93% for the multi-classification scenario. Besides, the training time of the proposed ensemble model is lower than the training time of benchmark techniques, demonstrating its efficiency and robustness.Keywords
Cite This Article
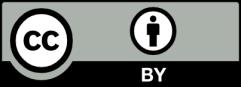
This work is licensed under a Creative Commons Attribution 4.0 International License , which permits unrestricted use, distribution, and reproduction in any medium, provided the original work is properly cited.