Open Access
ARTICLE
Abnormal Traffic Detection for Internet of Things Based on an Improved Residual Network
1 School of Computer Science and Technology, Xinjiang University, Urumqi, 830046, China
2 School of Cyber Science and Engineering, Jinling Institute of Technology City, Nanjing, 210000, China
3 College of Information Technology, Incheon National University, Incheon, 22012, Korea
* Corresponding Authors: Jia Wang. Email: ; Wei Hu. Email:
(This article belongs to the Special Issue: AI and Data Security for the Industrial Internet)
Computers, Materials & Continua 2024, 79(3), 4433-4448. https://doi.org/10.32604/cmc.2024.051535
Received 07 March 2024; Accepted 19 April 2024; Issue published 20 June 2024
Abstract
Along with the progression of Internet of Things (IoT) technology, network terminals are becoming continuously more intelligent. IoT has been widely applied in various scenarios, including urban infrastructure, transportation, industry, personal life, and other socio-economic fields. The introduction of deep learning has brought new security challenges, like an increment in abnormal traffic, which threatens network security. Insufficient feature extraction leads to less accurate classification results. In abnormal traffic detection, the data of network traffic is high-dimensional and complex. This data not only increases the computational burden of model training but also makes information extraction more difficult. To address these issues, this paper proposes an MD-MRD-ResNeXt model for abnormal network traffic detection. To fully utilize the multi-scale information in network traffic, a Multi-scale Dilated feature extraction (MD) block is introduced. This module can effectively understand and process information at various scales and uses dilated convolution technology to significantly broaden the model’s receptive field. The proposed Max-feature-map Residual with Dual-channel pooling (MRD) block integrates the maximum feature map with the residual block. This module ensures the model focuses on key information, thereby optimizing computational efficiency and reducing unnecessary information redundancy. Experimental results show that compared to the latest methods, the proposed abnormal traffic detection model improves accuracy by about 2%.Keywords
Cite This Article
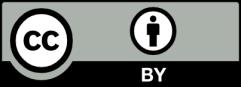