Open Access
ARTICLE
Learning Vector Quantization-Based Fuzzy Rules Oversampling Method
School of Mathematics and Physics, Hebei University of Engineering, Handan, 056038, China
* Corresponding Author: Litao Ma. Email:
Computers, Materials & Continua 2024, 79(3), 5067-5082. https://doi.org/10.32604/cmc.2024.051494
Received 06 March 2024; Accepted 09 May 2024; Issue published 20 June 2024
Abstract
Imbalanced datasets are common in practical applications, and oversampling methods using fuzzy rules have been shown to enhance the classification performance of imbalanced data by taking into account the relationship between data attributes. However, the creation of fuzzy rules typically depends on expert knowledge, which may not fully leverage the label information in training data and may be subjective. To address this issue, a novel fuzzy rule oversampling approach is developed based on the learning vector quantization (LVQ) algorithm. In this method, the label information of the training data is utilized to determine the antecedent part of If-Then fuzzy rules by dynamically dividing attribute intervals using LVQ. Subsequently, fuzzy rules are generated and adjusted to calculate rule weights. The number of new samples to be synthesized for each rule is then computed, and samples from the minority class are synthesized based on the newly generated fuzzy rules. This results in the establishment of a fuzzy rule oversampling method based on LVQ. To evaluate the effectiveness of this method, comparative experiments are conducted on 12 publicly available imbalance datasets with five other sampling techniques in combination with the support function machine. The experimental results demonstrate that the proposed method can significantly enhance the classification algorithm across seven performance indicators, including a boost of 2.15% to 12.34% in Accuracy, 6.11% to 27.06% in G-mean, and 4.69% to 18.78% in AUC. These show that the proposed method is capable of more efficiently improving the classification performance of imbalanced data.Keywords
Cite This Article
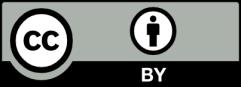