Open Access
ARTICLE
Weak Fault Feature Extraction of the Rotating Machinery Using Flexible Analytic Wavelet Transform and Nonlinear Quantum Permutation Entropy
1 College of Aeronautics and Astronautics, Taiyuan University of Technology, Taiyuan, 030024, China
2 Commercial Aircraft Corporation of China, Ltd., Shanghai, 200126, China
3 College of Physics and Electronic Engineering, State Key Laboratory of Quantum Optics and Quantum Optics Devices, Shanxi University, Taiyuan, 030006, China
* Corresponding Author: Lili Bai. Email:
(This article belongs to the Special Issue: Industrial Big Data and Artificial Intelligence-Driven Intelligent Perception, Maintenance, and Decision Optimization in Industrial Systems)
Computers, Materials & Continua 2024, 79(3), 4513-4531. https://doi.org/10.32604/cmc.2024.051348
Received 03 March 2024; Accepted 12 April 2024; Issue published 20 June 2024
Abstract
Addressing the challenges posed by the nonlinear and non-stationary vibrations in rotating machinery, where weak fault characteristic signals hinder accurate fault state representation, we propose a novel feature extraction method that combines the Flexible Analytic Wavelet Transform (FAWT) with Nonlinear Quantum Permutation Entropy. FAWT, leveraging fractional orders and arbitrary scaling and translation factors, exhibits superior translational invariance and adjustable fundamental oscillatory characteristics. This flexibility enables FAWT to provide well-suited wavelet shapes, effectively matching subtle fault components and avoiding performance degradation associated with fixed frequency partitioning and low-oscillation bases in detecting weak faults. In our approach, gearbox vibration signals undergo FAWT to obtain sub-bands. Quantum theory is then introduced into permutation entropy to propose Nonlinear Quantum Permutation Entropy, a feature that more accurately characterizes the operational state of vibration simulation signals. The nonlinear quantum permutation entropy extracted from sub-bands is utilized to characterize the operating state of rotating machinery. A comprehensive analysis of vibration signals from rolling bearings and gearboxes validates the feasibility of the proposed method. Comparative assessments with parameters derived from traditional permutation entropy, sample entropy, wavelet transform (WT), and empirical mode decomposition (EMD) underscore the superior effectiveness of this approach in fault detection and classification for rotating machinery.Keywords
Cite This Article
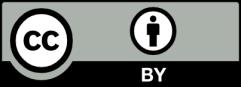
This work is licensed under a Creative Commons Attribution 4.0 International License , which permits unrestricted use, distribution, and reproduction in any medium, provided the original work is properly cited.