Open Access
ARTICLE
Deep Learning-Based ECG Classification for Arterial Fibrillation Detection
1 Faculty of Computer Science & Information Technology, The Superior University, Lahore, 54000, Pakistan
2 Intelligent Data Visual Computing Research (IDVCR), Lahore, 54000, Pakistan
3 Department of Computer Science, National University of Technology, Islamabad, 45000, Pakistan
4 Information Systems Department, College of Computer and Information Sciences, Imam Mohammad Ibn Saud Islamic University (IMSIU), Riyadh, 11432, Saudi Arabia
5 Department of Computer Science, Applied College, Taibah University, Medina, 42353, Saudi Arabia
* Corresponding Authors: Muhammad Sohail Irshad. Email: ; Sheeraz Akram. Email:
(This article belongs to the Special Issue: Deep Learning in Medical Imaging-Disease Segmentation and Classification)
Computers, Materials & Continua 2024, 79(3), 4805-4824. https://doi.org/10.32604/cmc.2024.050931
Received 23 February 2024; Accepted 25 April 2024; Issue published 20 June 2024
Abstract
The application of deep learning techniques in the medical field, specifically for Atrial Fibrillation (AFib) detection through Electrocardiogram (ECG) signals, has witnessed significant interest. Accurate and timely diagnosis increases the patient’s chances of recovery. However, issues like overfitting and inconsistent accuracy across datasets remain challenges. In a quest to address these challenges, a study presents two prominent deep learning architectures, ResNet-50 and DenseNet-121, to evaluate their effectiveness in AFib detection. The aim was to create a robust detection mechanism that consistently performs well. Metrics such as loss, accuracy, precision, sensitivity, and Area Under the Curve (AUC) were utilized for evaluation. The findings revealed that ResNet-50 surpassed DenseNet-121 in all evaluated categories. It demonstrated lower loss rate 0.0315 and 0.0305 superior accuracy of 98.77% and 98.88%, precision of 98.78% and 98.89% and sensitivity of 98.76% and 98.86% for training and validation, hinting at its advanced capability for AFib detection. These insights offer a substantial contribution to the existing literature on deep learning applications for AFib detection from ECG signals. The comparative performance data assists future researchers in selecting suitable deep-learning architectures for AFib detection. Moreover, the outcomes of this study are anticipated to stimulate the development of more advanced and efficient ECG-based AFib detection methodologies, for more accurate and early detection of AFib, thereby fostering improved patient care and outcomes.Keywords
Cite This Article
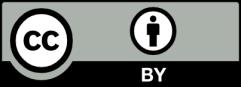
This work is licensed under a Creative Commons Attribution 4.0 International License , which permits unrestricted use, distribution, and reproduction in any medium, provided the original work is properly cited.