Open Access
ARTICLE
GCAGA: A Gini Coefficient-Based Optimization Strategy for Computation Offloading in Multi-User-Multi-Edge MEC System
1 School of Computing, Xi’an Shiyou University, Xi’an, 710065, China
2 Product Center, Wingtech Technology (Wuxi) Co., Wuxi, 214028, China
* Corresponding Author: Qiuchao Dai. Email:
Computers, Materials & Continua 2024, 79(3), 5083-5103. https://doi.org/10.32604/cmc.2024.050921
Received 22 February 2024; Accepted 10 May 2024; Issue published 20 June 2024
Abstract
To support the explosive growth of Information and Communications Technology (ICT), Mobile Edge Computing (MEC) provides users with low latency and high bandwidth service by offloading computational tasks to the network’s edge. However, resource-constrained mobile devices still suffer from a capacity mismatch when faced with latency-sensitive and compute-intensive emerging applications. To address the difficulty of running computationally intensive applications on resource-constrained clients, a model of the computation offloading problem in a network consisting of multiple mobile users and edge cloud servers is studied in this paper. Then a user benefit function EoU (Experience of Users) is proposed jointly considering energy consumption and time delay. The EoU maximization problem is decomposed into two steps, i.e., resource allocation and offloading decision. The offloading decision is usually given by heuristic algorithms which are often faced with the challenge of slow convergence and poor stability. Thus, a combined offloading algorithm, i.e., a Gini coefficient-based adaptive genetic algorithm (GCAGA), is proposed to alleviate the dilemma. The proposed algorithm optimizes the offloading decision by maximizing EoU and accelerates the convergence with the Gini coefficient. The simulation compares the proposed algorithm with the genetic algorithm (GA) and adaptive genetic algorithm (AGA). Experiment results show that the Gini coefficient and the adaptive heuristic operators can accelerate the convergence speed, and the proposed algorithm performs better in terms of convergence while obtaining higher EoU. The simulation code of the proposed algorithm is available: .Keywords
Cite This Article
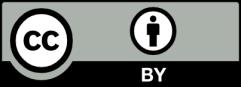
This work is licensed under a Creative Commons Attribution 4.0 International License , which permits unrestricted use, distribution, and reproduction in any medium, provided the original work is properly cited.