Open Access
ARTICLE
Multiscale and Auto-Tuned Semi-Supervised Deep Subspace Clustering and Its Application in Brain Tumor Clustering
1 School of Artificial Intelligence and Computer Science, Jiangnan University, Wuxi, 214122, China
2 Department of Medical Imaging, The Changshu Affiliated Hospital of Soochow University, Suzhou, 215500, China
3 Department of Neurosurgery, The Changshu Affiliated Hospital of Soochow University, Changshu, 215500, China
4 Department of Scientific Research, The Changshu Affiliated Hospital of Soochow University, Suzhou, 215500, China
5 Changshu Key Laboratory of Medical Artificial Intelligence and Big Data, Suzhou, 215500, China
6 Department of Biomedical Engineering, Faculty of Engineering, University of Malaya, Kuala Lumpur, 50603, Malaysia
* Corresponding Author: Kaijian Xia. Email:
(This article belongs to the Special Issue: Advanced Artificial Intelligence and Machine Learning Frameworks for Signal and Image Processing Applications)
Computers, Materials & Continua 2024, 79(3), 4741-4762. https://doi.org/10.32604/cmc.2024.050920
Received 22 February 2024; Accepted 28 April 2024; Issue published 20 June 2024
Abstract
In this paper, we introduce a novel Multi-scale and Auto-tuned Semi-supervised Deep Subspace Clustering (MAS-DSC) algorithm, aimed at addressing the challenges of deep subspace clustering in high-dimensional real-world data, particularly in the field of medical imaging. Traditional deep subspace clustering algorithms, which are mostly unsupervised, are limited in their ability to effectively utilize the inherent prior knowledge in medical images. Our MAS-DSC algorithm incorporates a semi-supervised learning framework that uses a small amount of labeled data to guide the clustering process, thereby enhancing the discriminative power of the feature representations. Additionally, the multi-scale feature extraction mechanism is designed to adapt to the complexity of medical imaging data, resulting in more accurate clustering performance. To address the difficulty of hyperparameter selection in deep subspace clustering, this paper employs a Bayesian optimization algorithm for adaptive tuning of hyperparameters related to subspace clustering, prior knowledge constraints, and model loss weights. Extensive experiments on standard clustering datasets, including ORL, Coil20, and Coil100, validate the effectiveness of the MAS-DSC algorithm. The results show that with its multi-scale network structure and Bayesian hyperparameter optimization, MAS-DSC achieves excellent clustering results on these datasets. Furthermore, tests on a brain tumor dataset demonstrate the robustness of the algorithm and its ability to leverage prior knowledge for efficient feature extraction and enhanced clustering performance within a semi-supervised learning framework.Graphical Abstract
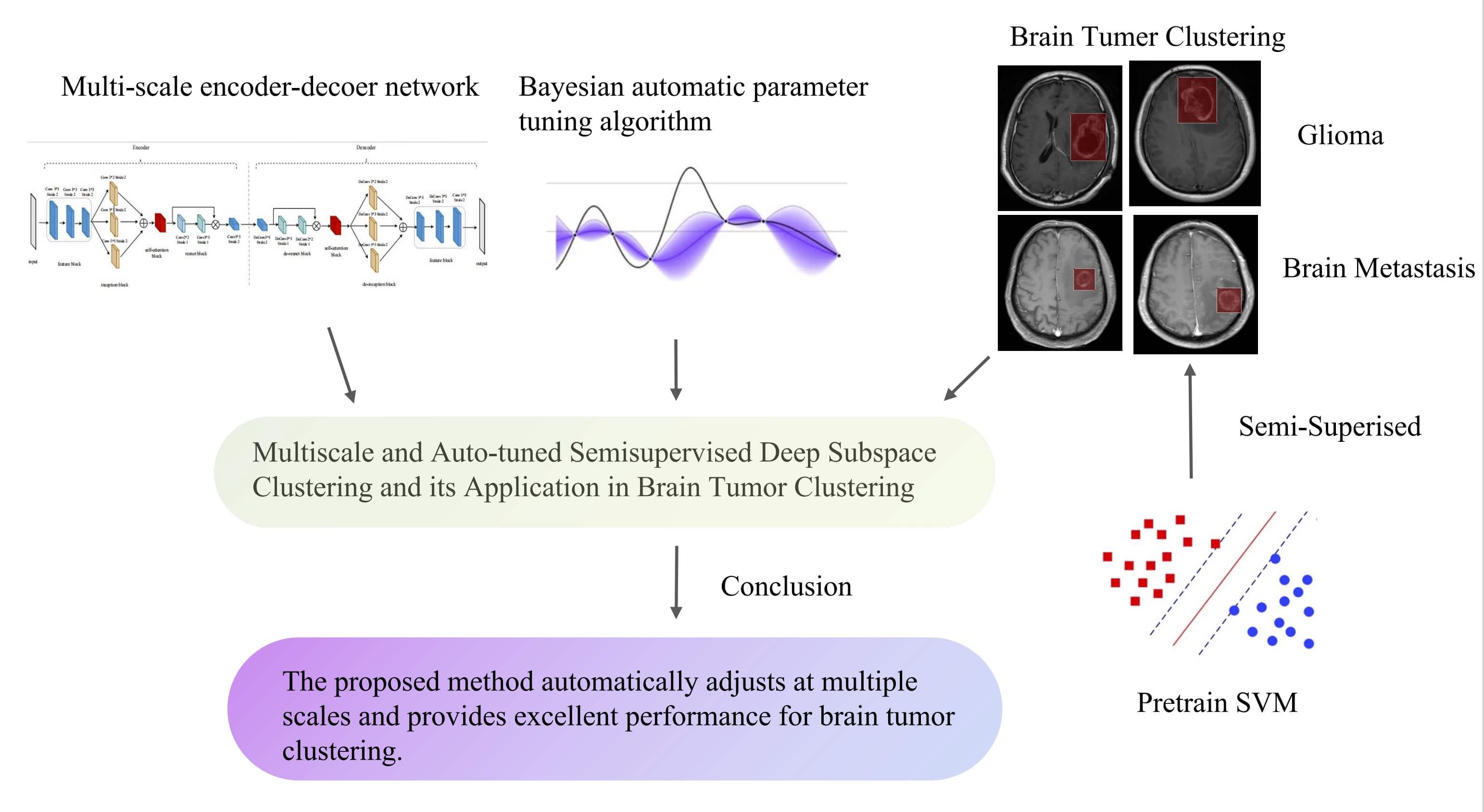
Keywords
Cite This Article
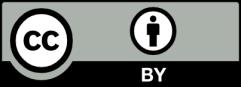