Open Access
ARTICLE
Fine-Grained Ship Recognition Based on Visible and Near-Infrared Multimodal Remote Sensing Images: Dataset, Methodology and Evaluation
Department of Aerospace Science and Technology, Space Engineering University, Beijing, 101416, China
* Corresponding Author: Min Hu. Email:
(This article belongs to the Special Issue: Multimodal Learning in Image Processing)
Computers, Materials & Continua 2024, 79(3), 5243-5271. https://doi.org/10.32604/cmc.2024.050879
Received 21 February 2024; Accepted 22 April 2024; Issue published 20 June 2024
Abstract
Fine-grained recognition of ships based on remote sensing images is crucial to safeguarding maritime rights and interests and maintaining national security. Currently, with the emergence of massive high-resolution multi-modality images, the use of multi-modality images for fine-grained recognition has become a promising technology. Fine-grained recognition of multi-modality images imposes higher requirements on the dataset samples. The key to the problem is how to extract and fuse the complementary features of multi-modality images to obtain more discriminative fusion features. The attention mechanism helps the model to pinpoint the key information in the image, resulting in a significant improvement in the model’s performance. In this paper, a dataset for fine-grained recognition of ships based on visible and near-infrared multi-modality remote sensing images has been proposed first, named Dataset for Multimodal Fine-grained Recognition of Ships (DMFGRS). It includes 1,635 pairs of visible and near-infrared remote sensing images divided into 20 categories, collated from digital orthophotos model provided by commercial remote sensing satellites. DMFGRS provides two types of annotation format files, as well as segmentation mask images corresponding to the ship targets. Then, a Multimodal Information Cross-Enhancement Network (MICE-Net) fusing features of visible and near-infrared remote sensing images, has been proposed. In the network, a dual-branch feature extraction and fusion module has been designed to obtain more expressive features. The Feature Cross Enhancement Module (FCEM) achieves the fusion enhancement of the two modal features by making the channel attention and spatial attention work cross-functionally on the feature map. A benchmark is established by evaluating state-of-the-art object recognition algorithms on DMFGRS. MICE-Net conducted experiments on DMFGRS, and the precision, recall, mAP0.5 and mAP0.5:0.95 reached 87%, 77.1%, 83.8% and 63.9%, respectively. Extensive experiments demonstrate that the proposed MICE-Net has more excellent performance on DMFGRS. Built on lightweight network YOLO, the model has excellent generalizability, and thus has good potential for application in real-life scenarios.Keywords
Cite This Article
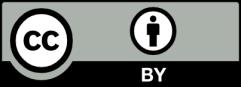
This work is licensed under a Creative Commons Attribution 4.0 International License , which permits unrestricted use, distribution, and reproduction in any medium, provided the original work is properly cited.