Open Access
ARTICLE
Vehicle Abnormal Behavior Detection Based on Dense Block and Soft Thresholding
1 School of Information Science and Technology, North China University of Technology, Beijing, 100144, China
2 School of Electrical and Control Engineering, North China University of Technology, Beijing, 100144, China
* Corresponding Author: Yuanyao Lu. Email:
(This article belongs to the Special Issue: Multimodal Learning in Image Processing)
Computers, Materials & Continua 2024, 79(3), 5051-5066. https://doi.org/10.32604/cmc.2024.050865
Received 20 February 2024; Accepted 08 May 2024; Issue published 20 June 2024
Abstract
With the rapid advancement of social economies, intelligent transportation systems are gaining increasing attention. Central to these systems is the detection of abnormal vehicle behavior, which remains a critical challenge due to the complexity of urban roadways and the variability of external conditions. Current research on detecting abnormal traffic behaviors is still nascent, with significant room for improvement in recognition accuracy. To address this, this research has developed a new model for recognizing abnormal traffic behaviors. This model employs the R3D network as its core architecture, incorporating a dense block to facilitate feature reuse. This approach not only enhances performance with fewer parameters and reduced computational demands but also allows for the acquisition of new features while simplifying the overall network structure. Additionally, this research integrates a self-attentive method that dynamically adjusts to the prevailing traffic conditions, optimizing the relevance of features for the task at hand. For temporal analysis, a Bi-LSTM layer is utilized to extract and learn from time-based data nuances. This research conducted a series of comparative experiments using the UCF-Crime dataset, achieving a notable accuracy of 89.30% on our test set. Our results demonstrate that our model not only operates with fewer parameters but also achieves superior recognition accuracy compared to previous models.Keywords
Cite This Article
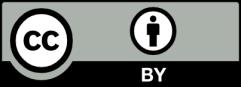
This work is licensed under a Creative Commons Attribution 4.0 International License , which permits unrestricted use, distribution, and reproduction in any medium, provided the original work is properly cited.