Open Access
ARTICLE
Exploring Multi-Task Learning for Forecasting Energy-Cost Resource Allocation in IoT-Cloud Systems
1 Department of Computer Engineering and Information, College of Engineering, Prince Sattam Bin Abdulaziz University, Wadi Al-Dawasir, 11991, Saudi Arabia
2 Department of Computer Engineering, College of Computer Science and Engineering, Taibah University, Madinah, 42353, Saudi Arabia
3 Department of Creative Technologies, Air University Islamabad, Islamabad, 44000, Pakistan
* Corresponding Author: Mohammad Aldossary. Email:
Computers, Materials & Continua 2024, 79(3), 4603-4620. https://doi.org/10.32604/cmc.2024.050862
Received 20 February 2024; Accepted 22 April 2024; Issue published 20 June 2024
Abstract
Cloud computing has become increasingly popular due to its capacity to perform computations without relying on physical infrastructure, thereby revolutionizing computer processes. However, the rising energy consumption in cloud centers poses a significant challenge, especially with the escalating energy costs. This paper tackles this issue by introducing efficient solutions for data placement and node management, with a clear emphasis on the crucial role of the Internet of Things (IoT) throughout the research process. The IoT assumes a pivotal role in this study by actively collecting real-time data from various sensors strategically positioned in and around data centers. These sensors continuously monitor vital parameters such as energy usage and temperature, thereby providing a comprehensive dataset for analysis. The data generated by the IoT is seamlessly integrated into the Hybrid TCN-GRU-NBeat (NGT) model, enabling a dynamic and accurate representation of the current state of the data center environment. Through the incorporation of the Seagull Optimization Algorithm (SOA), the NGT model optimizes storage migration strategies based on the latest information provided by IoT sensors. The model is trained using 80% of the available dataset and subsequently tested on the remaining 20%. The results demonstrate the effectiveness of the proposed approach, with a Mean Squared Error (MSE) of 5.33% and a Mean Absolute Error (MAE) of 2.83%, accurately estimating power prices and leading to an average reduction of 23.88% in power costs. Furthermore, the integration of IoT data significantly enhances the accuracy of the NGT model, outperforming benchmark algorithms such as DenseNet, Support Vector Machine (SVM), Decision Trees, and AlexNet. The NGT model achieves an impressive accuracy rate of 97.9%, surpassing the rates of 87%, 83%, 80%, and 79%, respectively, for the benchmark algorithms. These findings underscore the effectiveness of the proposed method in optimizing energy efficiency and enhancing the predictive capabilities of cloud computing systems. The IoT plays a critical role in driving these advancements by providing real-time data insights into the operational aspects of data centers.Keywords
Cite This Article
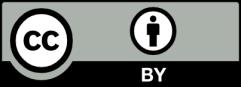
This work is licensed under a Creative Commons Attribution 4.0 International License , which permits unrestricted use, distribution, and reproduction in any medium, provided the original work is properly cited.